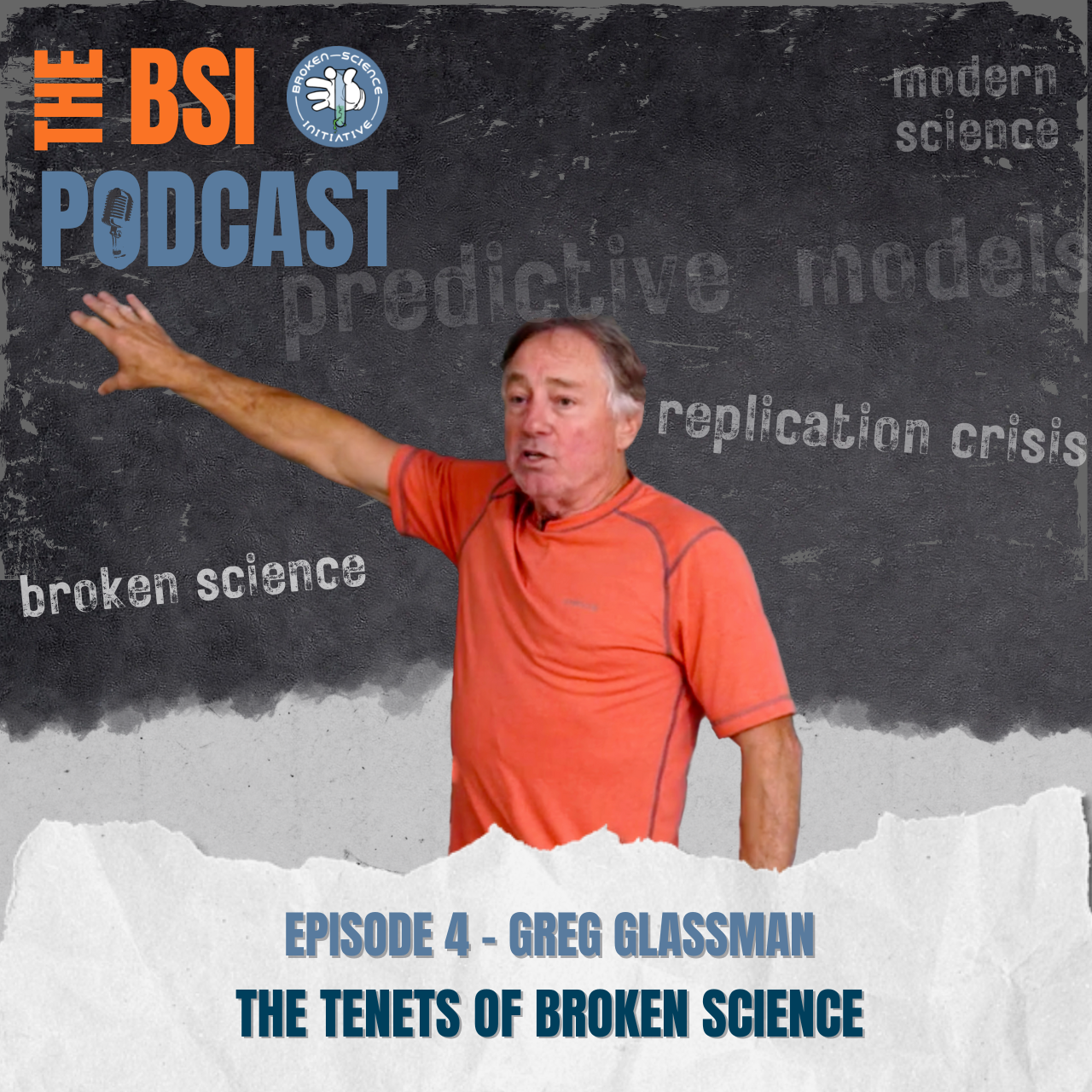
Ep 4: Greg Glassman – Breaking Down Broken Science
Science, once a beacon of objectivity, has become marred by corruption and misuse. At BSI, we are on a mission to unravel the tyranny of broken science and those who exploit it. In this week’s episode, we hear from Greg Glassman as he dives deep into the issues of broken science and addresses the consequences of research that will not replicate. This talk was recorded over a year ago in Santa Cruz California. Glassman, utilizing the chalkboards at the old CrossFit HQ, outlines the objectives of The Broken Science Initiative.
Transcript
Emily: Welcome to the Broken Science Podcast, where we consider what happens when predictive value is replaced by consensus in science.
This week on the Broken Science podcast, we’re featuring a talk Greg Glassman gave at the old CrossFit HQ building in Santa Cruz, California, over a year ago. This one wasn’t actually done before a public audience or really an audience at all. There were just a handful of us there. And he wanted to use the old chalkboards that he’d given so many talks on in the past to outline the project and some of the key concepts and criteria that we think are really fundamental for people to know and reasons why this project was launched in the first place.
I hope you enjoy it.
Greg Glassman: The Purpose here is to introduce the broken science initiative. Emily Kaplan is my partner in that effort and Clark Reed as well. In announcing it, I would expect to be asked, what’s an initiative? What is the initiative? And, what science is broken and what manner is it broken?
And I think we can do that quickly and simply here. At least that’s going to be the effort. Let’s just start with the initiative is to explore and discuss broken science and commit resources to that. I think that in this age, that is of particular importance in that, we are currently surrendering freedoms, to tyrants that are using, broken science as their primary tool to erode rights.
And what it is, that’s broken, this is simple. In fact, the hope in the initiative is that we’d like to free people from the tyranny of broken science and the tyrants that use it. And I think that can readily be done. What I mean by broken is I’m talking about the science that won’t replicate.
And this is a university science. It’s not a problem with industry. This isn’t a problem that Elon has. It is a problem that Stanford has. And there’s, I want to call your attention to a paper, Most Research Findings Are False. It was written by John Ioannidis at Stanford. He’s a, an esteemed medical researcher in the Department of Mathematics Public Health, Infectious Disease.
And and the title pretty much says the thing here, Most Research Findings Are False, meaning they won’t replicate. Sounds to me like about half, or more maybe. At least half, but there’s another important bit of research that’s critical here. And Begley and Ellis, Ellis is from UT Southwestern in Dallas and Glenn Begley was a director of research at Amgen and the two of them, funded by Amgen, spent a billion dollars and took 10 years, took a decade, and they examined 53 preclinical studies in preclinical oncology and hematology, and these are studies on which the drug trials were based.
And Begley had noticed a poor track record in getting drugs successfully through clinical trials, and so became suspicious of the underlying science, and attempted to replicate these 53 studies, and found that only 11% of them would replicate. And that’s horrific. We still don’t know what won’t replicate and what will.
And one of the studies that didn’t replicate, I understand has been cited several thousand times more. And so you have good reason to question what the bedrock of oncology and hematology is. The fields of sociology, economics, psychology and medicine – unfortunately, one of our friends says it’s all of the ologies and medicine – suffer from this replication crisis.
And to point out how it broke, where it broke and who broke it, I think we first need to look at what science should be and how it is practiced in industry, away from academia. It’s got different characteristics. So let’s take a look at that.
I’ve got five facets of modern science. And they’re kind of fun. And if you hold onto this and pay attention and think about it, it jives well with common sense. In intuits nicely. I got these from a work, Evolution in Science, that my father had put together, Jeff Glassman, after retiring. He was head of research, internal research and development at Hughes the Aircraft Company.
But this is how it goes. This is what modern science is. One. It’s repository and source of objective knowledge. Repository and source of objective knowledge. Two, this knowledge is siloed in models, okay, and what a model does is it maps a current fact to a future, unrealized fact as a prediction. And validation comes through predictive power, solely through predictive power.
There’s no other, there’s no other road to validation. And in fact, the models come in four flavors. There’s conjecture, hypothesis, theory, and law. And those have been well delineated in terms of their predictive strength. And number five validation is independent of method. And that’s a critical point.
There isn’t a method that guarantees an outcome that replicates. And the deal is whether the theory comes from inspiration or perspiration, its validation comes solely through its predictive power. And so these are the five facets that are critical to modern science. And I want to just share some thoughts, some notes, maybe even slight editorial on each of these.
I’ve got five concepts related to that I want to share.
A: Predictive power is evidence for science’s objectivity, sole source of its reliability, and the demarcation between science and pseudoscience. And I could have just as easily said science and non science. But I picked pseudoscience because that’s what Karl Popper did. And, I bet we have a bone to pick with his approach, but the demarcation is important here. The thing that separates astronomy from astrology is that astronomy has predictive power. And so if someone says that there’s going to be an eclipse in 215 days and four hours, and it happens, that’s the kind of thing in astronomy, astrology has a little harder time with. Aries are happier and make better lovers. That’s a little different kind of thing where you may not be able to show any predictive power.
B: predictive power is determinant of a scientific model’s validity provides the basis for any rational trust of science. And there aren’t reasons outside of that.
C: Mapping a fact to a future fact as a prediction is an inductive argument where the first fact constitutes the premises and the second fact forms the conclusion. And so here we are, we’re clearly in the space of induction, and –
D: Induction arrives at conclusions with probability and not certainty.
You’ll see other definitions or distinctions demarcations from induction and deduction. I saw this one in Scientific American 25 or 30 years ago from Martin Gardner, and I thought it was spot on. It sheds light. You can get confused sometimes, not in the difference between induction and deduction, but what the significance of induction is. And this looking for universals from particulars and all that, I don’t find that particularly illuminating. But the idea that its conclusions come with probability and not certainty is a wonderful demarcation between induction and deduction.
E: all scientific knowledge is therefore, the fruit of induction validated by predictive power, which is a measure of probability. It’s worth saying again, all scientific knowledge is therefore the fruit of induction validated by predictive power, which is a measure of probability and I have a quote here from Laplace. Probability theory is the calculus of inductive reasoning.
And so the whole thing, the key to all of what we’re doing here in modern science, it has to do with prediction. It has to do with prediction. That is the demarcation. Now what’s happened, and we know where and who and when, the process, the mess starts in 1934 with Karl Popper.
I don’t like to talk about Popper, Kuhn, Lakatos, and Feyerabend, without talking about the Australian philosopher David Stove. He dedicated his entire philosophical career, the span of it, to correcting the errors that were made by these guys. And there are many of us that think he did an absolutely amazing job of that. It was very convincing. In fact, I was, and he did it with with three different books. One of them’s been published three or four times with different titles.
And people are surprised that it doesn’t catch on. It’s not an easy read, but it’s an important read, and it’s Popper and After. But after reading Stove, and it took me years to get through his work, I left perfectly convinced that science found its grounding, its logic in probability theory. but I thought that I should be able to then go into probability theory and find someone that had come to those same conclusions.
And I had, in fact, and that was E. T. Jaynes. But I mentioned David Stove because his treatment of these philosophers should form the backbone of any correction or coherent philosophy of science. It’d be nice to have a philosophy of science that, that didn’t make scientists laugh and what we currently have does.
How pervasive is the Popper, Kuhn, Lakatos, Feyerabend what Stove called the irrationalists? How accepted or ingrained is their view? Kuhn’s structure of scientific revolutions is the most cited work, book in all of the academic literature. And Kuhn is cited second only to Lenin, Vladimir Lenin, I think it is. Is that right? Is it Lenin? I think so.
And what Popper did was he didn’t have a definition of science, which I found troublesome, but he did offer a demarcation. And when you look up Karl Popper, it says he’s most known for his demarcation. And in fact, his demarcation of falsification was built into, is part of, the Daubert decision of the U. S. Supreme Court that describes, defines, what it is to have a scientific standing in legal cases, in the law. And what he did is, it was important to him that it be deductive, the basis for science. And he picked falsification because the plan was to use like a modus tollens, iterations of modus tollens to lead where you want to go.
And that’s the if P then Q as a premise, and I got a second premise of not Q, therefore not P. And this is deductive and our null hypothesis, testing, statistic testing comes through this, is related to that and justified. But anyways, it leads to a mathematics and inferential mathematics that recognizes the probability of data given the hypothesis, but denies the meaning of the probability of a hypothesis. Which strikes an arrow right through the heart of the what is the chief method of validation, the only method, the only way that we validate a scientific model is through the probability of the hypothesis or the theory. And in all of these things, the null hypothesis statistical testing protocol of Fisher, Neyman, Pearson, it’s a hybrid of their approaches to inferential statistics. It’s this problem is only found in academia.
And what I’m getting to here is that this is the science that won’t replicate. It won’t replicate, and there is no system of validation. And for reference on this, I don’t think you can do better than to look at Trafimow and Briggs and Gigerenzer and some of the work that they did in conjunction with one another.
Very powerful. This relates to the problem with p values. Which there’s more written on that than you care to read. And this science that won’t replicate puts us in a position here. I’m going to go to the top board. I apologize for all the reading, but I didn’t want to leave anything out, I know I don’t have much time.
The deductive approach that denies the probability of a hypothesis and only looks at the probability of the data given the hypothesis, the null hypothesis testing, all of that leaves academic science with no measure, no metric for validation and offers instead the implicit yet false replication implication.
A validation through small p values in publication in journals esteemed by consensus. The science won’t replicate because it wasn’t designed to. Even the recognition of a crisis, in quotes, in non replicating science presupposes that it should replicate, suggesting an unspoken, maybe subconscious admission of the primacy of prediction in validating scientific models.
You could say, why, what’s the problem that it doesn’t replicate? It’s in the journal, it’s in the high impact journal. It was cited an amazing number of times, right? And it had good p values. What else are you looking for? I tell you, to be real, it needs to replicate. What would this look like in a, in terms of an offering?
I suppose you’d guide students to what to look at. First of all, I wouldn’t put much time into Popper, Kuhn, Lakatos, and Feyerabend. I could skim their work quickly, even though they wrote way too much. But I would fundamentally be able to dismiss them through the work of David Stove.
And he was he’s worth the study. This is not. And the point I’m making here is that Popper was dead wrong on the falsification.
Falsification. I think the first time I saw that, I think it was A. J. Ayers, the, one of the logical positivists, his logical positive is in one of the Vienna School. I think that was his requirement for a meaningful assertion. That it had to be a testable concept, at least in theory could be tested and it keeps things from seven angels to dancing on the head of a pin, it keeps that kind of thing from having meaning.
I would accept a demarcation of falsification if it were saying that’s a requirement for a meaningful statement, in the sense of a testable proposition. But it’s necessary for a scientific assertion or model, but it’s not sufficient. That’s the problem with it: necessary, but not sufficient.
And what I would do instead is I would study Laplace, Jeffreys and Cox. And in E. T. Jayne’s masterwork, Probability Theory, The Logic of Science, he goes into considerable detail as to how these three people created a probability logic. And this, in fact, is much of the strength and core of what’s known as a Bayesian inference today.
That’s the replication crisis in a nutshell, right there. This is broken science. I don’t think we’re going to fix it. I have no hopes of changing that system. There’s too much money, too much power, it would require a major revamp. But again, I do believe that we can protect any man, not every man, any man, anyone’s willing to listen and think.
I think we can protect them from the tyranny of bad science and its purveyors. Thanks. We’re done.
Emily: Thanks for listening. Don’t forget to follow along with all of our social media channels, as well as going to broken science. org and signing up for the newsletter, sharing with friends, interacting with the community and checking out all of the incredible resources we have there.
Which include show notes on each podcast episode, which is essentially a transcript of the show with links out to every reference mentioned. Our goal with doing that is to allow you all to fact check what we’re saying, as well as to allow you to continue to explore on your own any of these topics that interest you.
Also, if you found this episode helpful, we’d greatly appreciate you leaving a review. It tremendously helps us grow our audience and let other people know about the Broken Science Initiative. Thanks for joining us.
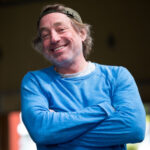