Long before AI entered our daily vocabulary, physicist and mathematician E.T. Jaynes was already pondering a reality where machines could reason like humans. His posthumously published Probability Theory: The Logic of Science gives us an outline of Jaynes’ thought experiment, a machine capable of plausible reasoning, stepping beyond cold, deductive rules to engage in inductive thought. Read through the lens of 2024, where AI models like ChatGPT have become part of daily life, Jaynes’ words feel almost prophetic.
“Models have practical uses of a quite different type. Many people are fond of saying, ‘They will never make a machine to replace the human mind—it does many things which no machine could ever do.’ A beautiful answer to this was given by J. von Neumann in a talk on computers given in Princeton in 1948, which the writer was privileged to attend. In reply to the canonical question from the audience [‘But of course, a mere machine can’t really think, can it?’], he said: “You insist that there is something a machine cannot do. If you will tell me precisely what it is that a machine cannot do, then I can always make a machine which will do just that!”
—ET Jaynes
Jaynes spoke of a machine designed to assist us in reasoning, an idealized robot that would help humans make sense of overwhelming amounts of data. He posited, “The only real limitations on making ‘machines which think’ are our own limitations in not knowing exactly what ‘thinking’ consists of.” In many ways, this captures the core of our modern relationship with AI. Models like ChatGPT help us make sense of language, data, and, occasionally creativity. They mimic reasoning, sometimes with striking accuracy, but are still bound by the very limitations Jaynes foresaw.
At the heart of Jaynes’ thinking is a concept that deeply inspires core principles of The Broken Science Initiative: predictive power.
“Predictive power is evidence of and reason for sciences’ objectivity, the sole source of science’s reliability and the demarcation between science and pseudoscience. Predictive power as determinant of a scientific model’s validity provides the basis for any rational trust of science.”
—Greg Glassman BSI manifesto
Jaynes’ imagined robot, then, was not just a tool for reasoning but a vehicle for basing predictive models on real-world data, helping us bridge the gap between what we know and what we have yet to discover.
“In order to direct attention to constructive things and away from controversial irrelevancies, we shall invent an imaginary being. Its brain is to be designed by us, so that it reasons according to certain definite rules. These rules will be deduced from simple desiderata which, it appears to us, would be desirable in human brains; i.e. we think that a rational person, should he discover that he was violating one of these desiderata, would wish to revise his thinking.”
—ET Jaynes
While Jaynes’ robot worked within the pristine confines of logic, AI today functions amid the ambiguity of human language and the complexity of data that is often incomplete or biased. And this is where the cracks start to show. For example, the rampant use of AI in scientific publishing, a world that should be grounded in the predictive power of reliable models but is increasingly marred by fraudulent papers. Just recently, Wiley, one of the world’s leading academic publishers, had to shut down 19 journals after they were compromised by fraudulent activity, much of it fueled by AI-generated content. This is a tragic twist in the tale of technology, one where the very tools with such potential to aid scientific progress have instead contributed to its corrosion.
Jaynes, had he lived to see this, might have been horrified. His thought experiment robot was designed to model plausible reasoning and arrive at truth through a disciplined application of probability theory. Yet, here we are, where AI has been used not just to advance knowledge but to fabricate it. A recent study found that over 1% of all scientific papers published in 2022, about 60,000 papers, were likely written by AI models. This flood of AI-generated content has led to a sharp rise in retracted papers, with Nature reporting over 10,000 retractions in 2023 alone, a new and troubling record.
Jaynes was clear about the power of reasoning machines, but he also recognized their limitations. Machines, he argued, can only do what we are able to describe in detail. Human reasoning may seem simple because we do it every day almost without much thought, but as Jaynes points out, “this reasoning process goes on unconsciously, almost instantaneously, and we conceal how complicated it really is by calling it common sense.” The complexity of plausible reasoning, intuition, or even basic common sense is something we haven’t fully unraveled, which implies we can’t fully teach it to AI. As long as we’re unable to clearly define how these processes work, AI models will struggle to replicate “common sense” with accuracy.
Wiley’s confession that its journals had been compromised by fraudulent articles, including studies on critical medical issues like drug resistance in newborns, is a stark reminder of the dangers. These fraudulent activities undermine public trust not only in academic institutions but in science itself.
Ironically, in response to this crisis, AI tools are now being developed to detect fake papers, machines policing the very fraud they enabled. It’s a disheartening cycle, one that raises critical questions about the role of technology in modern science.
The rot exposed at Wiley and elsewhere calls for a deeper, more human response. Some scholars are now pushing to move away from an overreliance on traditional peer review and toward face-to-face debates, where experts are held accountable and must actively defend their data and conclusions. The rise of AI in scientific research has laid bare the risks of placing too much trust in models without sufficient human oversight. As we grapple with the fallout of fraudulent research, it becomes clear that Jaynes’ vision of a truly thinking machine remains elusive. While AI can mimic reasoning, true scientific inquiry demands more—rigor, transparency, and, as the Broken Science Initiative insists, a relentless commitment to predictive power as the foundation of scientific validity.
In the end, Jaynes’ robot, much like today’s AI, is a tool—a powerful one, but one that is only as good as the minds that wield it. If we hope to realize the potential of these machines, we must do more than build models that map facts to predictions. We must ensure that those predictions are tied to real-world observations, grounded in reality, and subjected to the kind of scrutiny that only the human mind can provide.
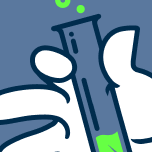
Support the Broken Science Initiative.
Subscribe today →
One Comment
Leave A Comment
You must be logged in to post a comment.
recent posts
Expanding Horizons: Physical and Mental Rehabilitation for Juveniles in Ohio
A reassessment of insulin sensitivity and insulin resistance
This is an excellent article. It is scary to think about the biases that can be in places such as ChapGPT.