Summary
William Briggs expands on Greg Glassman’s discussion about broken science by providing examples of scientific misconduct. He focuses on an experiment conducted by Nate Brena and a group of researchers, which serves as an illustration of such misconduct. The experiment involved distributing identical data to multiple research groups and assigning them the task of investigating whether immigration has an impact on public support for government provision of social policies. Briggs underscores the significance of understanding causality in scientific inquiry and the pursuit of uncovering the true essence of phenomena. Notably, approximately 25% of the models generated by the researchers concluded that immigration has a negative effect on policy support, while another 25% found the opposite. Intriguingly, all of these results were deemed statistically significant. However, Briggs raises concerns about the validity of these findings, as there should ideally be only one correct answer. He suggests that the prevalence of conflicting outcomes and the absence of a definitive solution may have led many scientific studies astray.
TRANSCRIPT
William Briggs: Well,
I’m gonna do a little experiment on this here. I’m gonna see how two talks
about statistics after you eat dinner aids in digestion. Everybody, everybody
loves statistics class, right? I mean, that’s the one thing that you look back
on your time in college about the grand times you had in statistics and
memorizing all those formulas and stuff.
I wanna, I’m basically gonna echo everything Greg just said.
I’m gonna say the same thing in just different words. I, I can’t even, I can’t
beat what Greg said, but I wanna, I want to just give you some more examples
along the same lines he did. So the first thing I want to tell you is about an
experiment that this guy Nate Brena and a bunch of other people did. There was
maybe a hundred authors on this paper, and this is a fascinating experiment for
us because it’s an experiment about experiments. What they did was they handed
out identical data, identical data, to a large number of researchers, large
number of groups of researchers, and they asked them to solve the same
identical question, to investigate the same question.
I’m gonna read it to you because I, I can never remember it.
The question was, would immigration reduce or increase public support for
government provision of social policies? I think it’s a little difficult to
remember. It’s hard to keep all this stuff in mind, and I want to give you some
other examples.
So I want to just classify this as, does X affect y, does X
affect y. X, immigration, affect Y, support for certain policies. Now that’s
causal language. Does X affect Y? And I think that science is about, cause. The
fundamental goal of science, of course, is to understand nature, to understand
exactly what nature is.
And that means understanding exactly what’s going on. And that
means understanding what the causes are. And if you truly know what the causes
are, you can make those predictions that we’re talking about. So that’s what
they did in this experiment. It was just exactly the right thing to do. Now
they sent all this out and I can’t stress enough.
This is identical data. Everybody had the exact same data and
they were asked the same exact question, but they were left free to investigate
it. However they wished with any set of modeling tools that they wished about a
quarter of the models came back. I should say they were first able to answer
this question, does X affect Y, yes or no?
And maybe we don’t have enough data to tell. Only one group of
researchers came back and said they could not tell. Everybody else provided a
definite answer. One group, one quarter of the, of the models that came back
said, yes, X affects Y negatively. More X, less Y, more X, less y, more
immigration, less support for these public policies.
And all of these results, this quarter of results were
significant. That’s the word we’re gonna come back to, is significant. And they
were allowed to give an estimate of the, the effect size, the, the strength of
the association between these two things. And all of them were significant, but
some of them were very strongly significant.
And some were just less so, but they were all still
significant. Now maybe you see this coming, but another quarter of the models.
Answered completely oppositely, they said, yes, X affects Y, but positively,
more X, more Y, more immigration, more support for these policies, completely
diametrically opposed.
And just like the first group, all of the, the quarter in this
group said, yes, it’s significant. Our results are significant. So you have two
groups, a large number of groups, and they’re at war with each other, every one
of them providing a definite answer that left about half the models that left
about half the models, and those were split roughly in half two.
Some of them said that X affects Y negatively and some about X
affects Y positively, but they weren’t quite significant, but they were still
sure of their answers. Now, this should sound wrong to you. This, if, if you’re
thinking this, this absolutely must think wrong. There’s only one right answer.
I don’t know what the right answer is.
And the answer might even be that there is no association
whatsoever in the one group that did not want to turn in a model. They were the
right ones. I don’t know. But this is the interesting thing about this is that
because there can be one only right answer, many people have gone wrong. A lot
of science has gone wrong.
But every model that was turned in was science. And as you
heard from Greg, we have to follow the science, don’t we? We’re all told to
trust the science. Follow the science, but whose science? So that’s what we’re
gonna talk about a little bit tonight. Now Well, these models, this was a, a
sociological question.
We already had a question about some of the weaknesses in
sociology and other fields like psychology and education and so forth. And it’s
not really too surprising. These fields are, there’s more controversy and so
forth, and perhaps more error because look of the hideous complexity of the
subject, people’s behavior and groups of people’s behavior, that’s really
difficult to know what’s going on with groups of people or even just one
individual.
So, you know, when we’re talking about that kind of science,
sometimes we call it the soft sciences. So to investigate the philosophy of
science most people don’t think about these things and they instead go to what
they called the hard sciences, like physics and chemistry and so forth, and
they think, well, now here’s, we got real science happening and, and this kind
of stuff that happens in sociology and psychology doesn’t really happen in physics
or not that often.
Well it does physicist Sabine Hossenfelder in a Guardian
article, I think it was last year she gave this quote about the particle
physics, about the state of particle physics, and I wanna read it to you cuz
it’s important. She says, since the 1980s, I’m quoting her now, physicists have
invented an entire particle zoo whose inhabitants carry names like preons,
sfermions, dyons, Magnetic Monopoles, simps, wimps, wimpzillas, axions,
flaxions, erebons, Acceleron, cornucopions, giant magnons, maximons, macros,
wisps, fips, branons, skyrmions, chameleons, cuscutons, planckons, sterile
neutrinos, to name just a few.
Now the thing about end quote, the, the thing about that is
none of them turned out to be real. Not one. And there were many, many more. So
it’s not just so psychology and sociology and the, like, it’s physics too. Now.
She blames in part the idea of falsification because if as long as you’re
providing falsifiable propositions, why then you’re doing science.
But I think that’s part of it. I think the real thing is is
just human psychology. Physicists want to be the people who are in discovering
that new particle, so they get to name it and everything. So there’s great
motivation to better themselves by coming up with these kinds of things.
Still, there’s a, there’s a bright side to that because as I
said, none of those particles turned out to be true. So what they did is the
right thing. In the end. They invented all these things perhaps too gidley.
But, in the end they checked. Can we predict and see this stuff again happening
again? And it alters, turned out to be statistical anomalies.
Now, one thing I didn’t emphasize in the Bresno thing was the
number of models that were turned in, it was 1200. I wanted to save that little
tidbit. 1,200 miles. Actually it was a little bit more 1200 models. Now with
that being the case, we have to conclude, we’re forced to conclude. And looking
at the physicist did too, doing science is easy, creating models is easy.
Positing theories is, is easy. It’s real easy to do this. And
so that’s part of our problem right there. We’ve got so good at this that we’re
doing too much of it. And that’s and, and you and you think about what happens.
I only gave you just one example and, and Greg gave you a few and Brett
mentioned some at the very beginning.
What if we apply all of this to all of science? What do we
have? Well, we have that replication crisis we talked about. And it was just
funny we did, I didn’t talk to Brett about this beforehand, but he mentioned.
In the one set of study of medicine, they tried to, what they did is lot of
groups of people from economics and sociology and physics and all big name
fields, they wanted to know how good the results were holding up.
So they looked at the top papers written by the top people in
the top journals, and they recreated the experiments. They redid them. They
redid them, seeing how well they’re gonna hold up now, it doesn’t matter which
field you’re at, which branch of science you’re doing, the result remarkably
was about the same.
Only one quarter of the results were the same direction and
size as the original experiments. Didn’t matter the field, a few more were
about the same effect, but not the same strength of association. And the rest
absolutely failed. Now that’s very interesting. So It’s that same one quarter
we fund everywhere.
And that’s kind of, we think back to what we just talked about,
Brenna’s experiment. We got one quarter of the models saying yes, and one
quarter of the positive and one quarter of the models saying negative. I got
that one quarter in here again. So that’s interesting. It was a, I’ll give you
just a couple examples here.
You can go on and on about this all day. They’ve done a lot of
these replication studies. Right now. We, we’ve already talked about John
Ioannidis. He did, he looked in medicine and he looked at the creme de la creme
of papers in medicine, which is to say the most popular papers, the papers that
got the most ti citations, a thousand citations each or more had to be to, to
make his list.
He found 49 pa- incidentally, scientists, scientists love
talking about their h indexes, citation counts, impact factors, source
normalize impacts per paper. They discuss these metrics and the journal metrics
they publish again. I mean, they, they talk about these things more than
Instagram people talk about their likes.
This is, I think scientists invented social media. You, you
just listen to them talk and this is the kind of thing they talk about. Anyway,
Ioannidis found for that the top 49 papers, the absolute top of the papers, 16%
that replicated in the, in the same direction as the original.
The British Medical Journal in 2017. I’m not gonna bore you
with a lot of these results, but this one’s interesting. They looked at new and
improved cancer drugs. So in, in Europe it’s pretty much the same like in the,
here, in the United States, new drugs get have to be approved by the fda and
you have to submit certain statistical evidence and that kind of a thing.
Same thing in Europe. And so the drugs all met this test to be
released into the wild. And so they looked at them several years down the road
after being released. 39 drugs, cancer drugs. And after a while, only 35% of
the new drugs had any important effect. About a third, about two thirds had no
effect whatsoever. And this is the kicker right here. I’ll read you this. The
magnitude of the benefit on overall survival ranged from about one to six
months. That’s it. From all this science, all this work about an average of
three months. It’s not nothing, but it’s not a lot.
Now, Richard Horton of the Lancet, he was the editor of The
Lancet in 2015. He’s still the editor now. He said this, I wanna read you this.
The case against science is straightforward. Much of the scientific literature,
perhaps half may be simply untrue afflicted by studies with small sample sizes,
tiny effects, invalid exploratory analyses and flagrant conflicts of interest.
Together with an obsession for pursuing fashionable trends of
dubious importance, science has taken a turn toward darkness. This is the
lancets, that’s the top journal, perhaps New and England Journal of Medicine.
Maybe you can have a, a war there. Now that’s the, the half of science that’s
wrong. Or perhaps three quarters.
This is the best science. This is still the top side. Imagine
what it’s like in the lower tiers where we don’t have such you know, enthusiasm
for the science. Now, you might’ve heard of this. There was a, a guy named
Russell Funk, a bunch of others. They looked at the papers through the years.
This appeared in nature oh two, three months ago, and they looked at what is
called disruptive science.
This is genuinely groundbreaking, fundamental new science new
fields of endeavor and things like this. They looked at that across every
field, from physics to psychology, education, everything. It had plummeted
since 1960. It’s now down to almost nothing in every single field, in
absolutely every single field.
So it’s impossible to look across the science that we’re seeing
out there and think that all of it, or even most of it is any good or of any
real use. There’s a tremendous problem out there. And there, there’s no
symmetry here. Even if half of science is right and half of science is wrong,
the half that’s wrong takes much more energy and effort to, to combat and
battle, especially because science is now run by the bureaucracy largely.
I mean, they can control the, the mass amount of funds that
goes on, and the people in charge are able to look at this sea of science, good
and bad, and pick from it what they want. And they say, here we go. This is the
result I want. This is now the science. And like Greg says, you ever know, they
don’t say this is science.
They say the science, they say follow the science. That’s why
the, we need to lessen the, the, the, the amount of, of bad science that we
have. So how do we do that? Well, other people have looked at this in, in 1960,
across the entire world and all fields of science. There were published about a
quarter, a million papers.
That has now gone up to about 8 million papers a year, and it’s
just skyrocketing. It’s still shooting north. So because of all the bad science
we’re seeing, because of this glut that we have, well, what’s the problem? The
problem is there’s too much science, there’s too much of it. There’s too much
money involved, there’s too much prestige involved.
There’s too many people doing science. There’s too many people
doing poor science, calling it good. And the solution to this is easy. It’s
really simple. Stop doing so much science, but that’s not gonna happen. You’re
not gonna see any politician come up there and say, you know what? All right.
You know what?
I’m for? Less funding for science education. So we’re not gonna
have so much of that. I’m gonna cut back on the budgets to the NSF and nih.
It’s not gonna happen. So we have to live with this. We have to fix it some way
or another. Alright, so now I’m not gonna go on about politics anymore. I wanna
know what counts as bad science and, and let’s think about that a little bit
and what produces it besides just the glut of it, what else produces it?
A lot of the reasons we already know about. A lot of the stuff
you guys know about, like peer review. It’s true. Scientists, you’ve heard it
all the time. Scientists must publish or perish. That’s an absolute fact. They
must publish. So that accounts for this glut of papers. They have to put
something out all the time.
And this is Richard Smith, now editor of the British Medical
Journal in 2015. He said if peer review were a drug, it would never get on the
market because we have lots of evidence of its adverse effects and don’t have
evidence of its benefit. It’s time to slaughter the sacred cow. It won’t be. It
won’t be it.
Peer review is too necessary to the journal system, which
scientists need for their for their bonafides to make their money. So, and we
can’t change that system. Fundamentally, the peer review it, it adds to the
surface of papers that we have and it, it, it guarantees a system. It guarantees
banality, it penalizes departure from consensuses.
It limits innovation. It drains time. Almost as much time. I
dunno, do we have a lot of working scientists here? Almost as much time as it
takes to write grants for not only must you publish, you have to provide
overhead for your dean, and if you don’t, you’re not gonna be able to keep your
job at a lot of major research universities.
So that’s a problem. Also, finally, we come to the philosophy
of science that’s ostensibly our, our job up here. And I, I wanted to start
with that, but I couldn’t, I didn’t think I could because a science is held in
such awe, great awe, which a lot of it’s justified, but a lot of it’s not, as
we’ve seen, and I had to at least kind of.
Weaken that awe that a lot of us feel before I can tell why I
think science goes bad in detail. So that’s what I want to do right now. Now,
again, I said at the beginning, I think science the job of science, the
fundamental job of science, the detail that Greg talked to you about, how to do
it, you know, the, the mechanics of it.
Of course, I agree with that, absolutely. But the, the
fundamental goal is to understand nature. And if we understand nature, we need
to understand cause Y and how and when X causes y. And that depends on a
philosophy of nature. You need to have a philosophy of nature. You need to have
a philosophy, uncertainty, and of what models and theories are and the like.
Now, there’s a lot of dispute in these areas and so forth. I’ll
just give you one. We can’t do all of that. So I’ll just give you one flavor.
There’s people out there who call themselves instrumentalists. They don’t think
causes the most important thing. They’re satisfied with statements. Like, if X
then why?
If X then y that’s different than X causes y. It sounds
similar, but it’s not quite the same. It only says that if X happens, then Y
will follow. Doesn’t say y doesn’t give you any real details. Y and it’s not
like, it’s not useful. It, it’s, it’s darn useful. You think about a a lady
getting on a plane, she has no idea how the engine in conjunction with the
wings and the fuselage work to get the plane to fly, but she knows the plane
does fly if x then why?
It’s not, it’s not completely useless or something. It’s a
first step. And scientists do that all the time, of course, by varying
conditions in their experiments just to see what’s happening. So that’s one
part of of a philosophy of science that’s useful, but doesn’t get to the core
of things, which is cause.
And so now what I want to do is lead us through six or seven
short examples. What I think are the, the main ways that science goes wrong by,
In attention to philosophy and not paying attention or paying heed to these
important philosophical differences. Now, I’m gonna start with, I think I the
easiest to explain and understand and to the most difficult.
I’m not gonna go through every possible way or anything like
that. Just the ones I think are the most productive of, of bad science. And we
do know that there’s lots of bad science. We’ve already seen that the number
one way is this X. The X we’ve been talking about is not, not measured, but a
proxy for X is, and everybody forgets the proxy.
So X is not measured. A proxy is, and everybody forgets it. This
one is extraordinarily popular in epidemiology. We’ve all heard from
epidemiologists these past three years. Without this fallacy, the the field
would be largely barren. Let me give you an example. PM 2.5 you might have
heard of it’s dust of a certain size.
It’s all the rage right now and everybody’s investigating it
for all. Its supposed deleterious effects. And you’ll see lots of papers pm 2.5
linked to or associated with heart disease or some such malady. And those are
kind of semi, sort of causal words, but not, they have technical definitions
which allow them escape clauses when it turns out not to be true, if it does.
And so they wanna say cause and they’re very happy to have
people infer cause from those words, but they don’t actually mean cause. So the
problem with all these studies, every study that I’ve seen anyway, is PM 2.5
intake is never measured, never measured. But they’ll, they’ll still say, PM
2.5 causes heart attacks or heart disease.
Now, why, how does that go bad? I’ll give you the example that
the American Heart Association we don’t need to know the names of these papers.
I have them if you, if you want to talk to me afterwards, touts for their main
study of the deleterious effects of PM 2.5. Here’s what they did in this
experiment.
They took the zip codes of where people live or rather their
primary residences. This is gonna be hard to follow. They got the, where they
live and they got the distance from a major highway from their home. Then they
have a model of pollution produced at that highway. Then they have a land use
model of how that, the PM 2.5 goes back to their primary residence, where it is
assumed that the model’s guess of the, the PM 2.5 at their residence was the
exposure to PM 2.5, not the intake.
Not the dose, the exposure. So we have in the end, I dunno
what’s going on. It’s not that PM 2.5 doesn’t cause heart disease. It may, it
may not, I don’t know. But the problem we have here is over certainty, vast
over certainty. There’s far too many steps in that causal chain to know what’s
going on. So that’s, oh, can I indulge you with can you indulge me?
But my absolute favorite example of this, this is, I, I tell
this one all the time, but it’s my favorite one. The Kennedy School. Harvard’s
Kennedy School. Some researchers claimed x causes y that attending a 4th of
July parade turns kids into Republicans
parade. Attendance was never measured. Instead, they measured
rainfall at the location of the residence of where the kids lived when they
were children. If it rained, they said, No. 4th of July parade could have
possibly taken place and so the kid didn’t go to one. Even if they were away at
their grandmother’s or something like this.
And if it was sunny or it didn’t rain, no precipitation could
have been cloudy. They said a parade happened and the kid went there with no
measurement and they used causal language. They used this, made all the papers.
It was on all the news. Couple years back, experiencing 4th of July and
childhood increases the likelihood that people identify with and vote for the
Republican party as adults.
Well, I’m a meteorologist by train. It never rains in San
Francisco in July. It should be a hotbed of Republicanism, right? Carver.
We’ll get to why that, why they came to that. Anyway. Number
two, the second most popular way that things go bad by not heating philosophy.
Y is not measured, but a proxy for Y is, and everybody forgets the proxy and
sometimes neither X or Y is measured. I call it the double epidemiologist
fallacy. These are my fanciful names.
You’re not gonna find them out in the literature or anything
like this. The CDC is a big user of this fallacy I’m afraid to say. That’s how
they talked themselves into mask mandates. In spite of a centuries worth of
studies, we have a centuries worth of studies showing masks do not slow or stop
the spread of respiratory viruses.
Starting from after the Spanish flu pandemic in 19 17, 2 years
later, they started doing these studies. We got studies all the way up through,
we have studies in operating rooms where in a major hospital in Britain, people
weren’t wearing, the surgeons weren’t wearing masks for like six weeks. No
difference between infection rates and everything.
On and on. And in 2020, March, 2020, there was a major
meta-analysis that came out. Coincidentally, the paper was in the works for
some time before the covid panic hit showing it was flu, not covid, cuz no one
knew of covid that time. Masks did nothing. Masks did nothing. But it was
ignored. It was just ignored.
So how did the CDC come to their belief that masks work? Well,
they looked at cases up until 2020. Cases meant people who were seriously ill
and sought or needed treatment and we, we differentiated them from infections.
But that, that all changed in the panic anyway. They looked at cases in
counties with and without mandates, or rather, they looked at rates of change of
case rates from county to county with and without mandates.
And they said, look at this statistical model we have here that
proves that mandates are good, mass mandates are good. It doesn’t work that
way. Neither X nor y has been measured when nobody was measured wearing a mask
or not. Nobody’s infection was measured or not, but they did do one study like
this.
Maybe you’ve heard about it. It was in Denmark. They, early on
in the panic they did in Denmark, they handed out an N 95 masks to thousands of
people and trained them in their use. They got free mask and trained in the use
and they and then another group went mask free thousands of people. And, and
they, at the end, they measured individuals.
Did you get an infection? Did you get an infection? Did you get
an infection? They measured this. That’s the exact way to do the experiment. No
difference in the groups. And that study was excoriated because it was not a
pleasing result. All right, number three, attempting to quantify the
unquantifiable.
Number three. Now, my favorite, one of my favorite novels of
all time is Little Big man. Don’t watch the movie. It’s terrible. It preaches
the exact opposite message of the book, but it tells the story of Jack Crab.
Maybe you’ve heard of the story. He was a, a white boy in 1850 in Wyoming,
what’s, what’s now Wyoming.
And he was adopted into a clan, a Cheyenne clan, and raised
among the Cheyenne. And many years later, he ended up back among the whites.
And he is amazed at all the quantification he sees. He said, that’s the kind of
thing you find out when you get back to civilization, what date it is, what
time of day, how many mile from Fort Leavenworth, and how much the settlers is
getting for tobacco there, and how many beers Flanagan drunk, and how many
times Hoffman did it With the Harlett numbers.
Numbers, I’ve forgotten how important they was. And I think
sometimes too important, I say this as a mathematician, statistician. Let me
ask everybody right now. This is important. We’re gonna do an experiment here.
How happy are you? This a statistics docs. It’s gonna be high. How happy are
you on a scale of negative 17.5 to e the natural number?
E cubed?
Well, all right. We could be serious and treat it. I I’m gonna
cut do it. I’ll say one to five. Now that makes it science. Now I can call it a
Likert scale because I’m quantifying it with, with, with integers instead. And
I’m not gonna call it the, I’m not gonna call it the happy score. I’m gonna
call it the Briggs instrument.
They call these questionnaires, instruments, and they attempt
to borrow with that name. The precision and rigor of things like the Werner Cas
and oscilloscopes and the like. Now suppose I pulled which hat looks more
bored. That’s kind of hard. Statistics. So let’s see this hat. This guys, these
are, these guys are smiling more than you over here.
So let’s suppose I asked this over the room over here. I asked
everybody the happiness, the patented, they’re all copyrighted, these, these
things. I, the patented Brigg score over here. And I asked over here, and I
found out that there was a difference in these scores. And can then I say that
the people seated to the speakers left are going to be happier than people
seated to the at the right for after dinner speakers, after dinner speeches.
Well, that’s how the science of this kind of thing is done.
That’s exactly how it’s done. It’s precisely how it’s done at anyway. What does
happy, what does happy mean? This is not one of the things I wanna read you.
This is source. I’ll just read you a couple of these. This is important.
Accepting, accidental.
A rem adapted, a advantageous, advisable, applicable, opposite,
appropriate Apropo app at ease, suspicious beaming. Be betide becoming bi.
That’s my favorite. Befitting. Bemused, beneficial, benign, benign and beaded.
Blessed beside beads pretty good, too blind, drunk, and so on, on and on. Each
of these is a genuine shade of happy.
Alright? Each of these is a genuine shade of happy, and it’s
not like I give this, this, this instrument out if I got a person who answered
the absolute top and a person who answered the absolute bottom. Yeah. I’m not
saying that this doesn’t say there’s some difference in happiness between these
people.
There might be, there probably is, but I don’t know what that
difference is. I don’t know what, what shade of happy these people have in
mind. And so how do I tell, well, the, the typical answer is to say, well, the
instrument has been validated. What does that mean? It doesn’t mean that I’d
send you oscilloscope out to some master thing and I’d calibrate it next to it.
No, it just means I give the questionnaire out to another group
of people and the answers come back about the same. That’s not true.
Validation, which can’t be had, which can’t be had, but we fool ourselves into
doing it. And why? Because that’s the only way we can use these statistical
methods.
Psychology is the one of the biggest abusers as we learn, cuz
without these quantifications, there’s no statistics to do on them, so we can’t
quantify the unquantifiable. All right, here’s the one of the big ones,
mistaking correlation for causation. No, everybody, every scientist knows that
you must not logically imply correlation.
A causation from correlation. Everybody’s heard that, right?
Correlation does not imply causation. Logically, just like confirmation bias
though, everybody’s heard of confirmation bias. That’s for the other guy. Now,
you could tell in Brenna’s group, I don’t know, they didn’t do a poll of these
people afterwards, but I bet you the people in the opposite camps each believed
that they had the right answer and the other people didn’t.
There must have been a lot of confirmation bias going on in
there, but nobody’s going to admit to it. Nobody who’s producing bad science is
gonna say, I’m the guy. It just doesn’t happen. That’s not how people work. So
why do people mistake correlation for causation? Well, the practice of
announcing measure of model fit to data, the practice of measuring model fit to
data.
The lancets Horton, we met Horton earlier. He said, our love of
significance pollutes the literature with many a statistical fairytale. This is
a big problem. Everybody knows about the problem, but nobody can wean
themselves off this. What does significance mean? Nothing to do with the
English word significance.
It means having a we p value. That’s all it means. And having a
we p v P value means it’s significant. There’s so many things wrong with
p-value. I mean, we could spend an hour talking about them or more. Should we?
No. All right. I’ll just leave it at this. When a we p value is found, it means
this, it means that the, there’s correlation in the data.
That’s what it means. But we can’t infer causation from
correlation. But that’s what’s done. That’s exactly what’s done. Everybody
infers causation from this correlation knowing they’re not supposed to do it,
but they do it anyway. They can’t stop themselves. Gerd, Gigerenzer who Greg
mentioned he, he calls it a, a ritual.
It’s it’s sciences ritual. It’s, it’s exactly that. We, we come
up here, we bless the data, we get the, we p value out. If you can’t get it, we
p value out of your data. You’re just not trying hard enough as Brenna’s
experiment proved. So we, we just have to abandon these things. But I’m not
gonna give you any, a lot of examples on that.
I’ll just say that it, it accounts for all those headlines. You
see, I gave this example out in Phoenix. Phoenix. You see these medical
headlines all the time. The headlines I like, these are actual headlines from
writing about actual medical trials and the like, one egg a day lowers your
risk of type two diabetes.
That’s causal language. You eat an egg a day, you’re gonna
lower your risk of diabetes. Another headline came out just a couple of months
afterwards. Eating one egg a day increases your risk of diabetes by 60%. Study
warns these things, we laugh, but they’re all over the place. And I united himself,
he wrote a paper in 2015, I think it was.
He looked at, he just grabbed a cookbook. He thought it’d be
fun and grabbed just 50 random ingredients and then went to PubMed to see how
many of them are associated with or linked to cancer. Just about every one of
them. Everything is, that’s, it’s too easy to find a correlation and this, the,
the causality of cancer is not just simply you’re gonna eat an egg or, or
anything like this, and you’re gonna develop cancer.
It doesn’t work that way. So model fit is of course it’s nice.
We need it. It’s a necessary criterion of that your theory’s working as it
should, but it’s not enough. We can’t do it. It you need to have much more than
this. You need to have predictive success. Aha. So we need to have that predictive.
And you’d be surprised, the physicist at least did that
predictive check of their models. They’re very enthusiastic about building
models and making them, but they at least went back and checked. They made
predictions with these things, found out they were poor models, and they
abandoned them. But that never happens.
And the other sciences people write a paper out in the world,
they go and they’re satisfied, they’re done. They never check it. That’s why we
have the replication crisis. That’s why so many of these papers fail. Only a
quarter of them coming back in the same shape they left. That’s how science
improves.
But physics is improved if it’s approved at all, just by,
because they’ve, they’ve done these kind of predictive checks. This is what
Jeff’s this is what Greg’s dad said. Jeffrey Glassman in a book. He wrote a
book on excellent book on how to do science that for that a lot of educators
should read and so forth.
Evolution in science. He says theories grow by eating. They’re
young. They feast on their own predictions. And I thought that was perfect. I
think that’s where Greg, Greg grew up learning this kind of stuff, but only the
good predictions. They choke and die on the bad ones as they should. But we
don’t have that.
We don’t have that in most fields. It’s just not existent. I
promised you it’s not there. All right, number five. We’re getting more in
depth and it’s more complicated as we go along these things, as I promised you,
so I wouldn’t. Blame you for not following all of this. It’s the multiplication
of uncertainties or rather the forgetting of multiplication, of uncertainties.
Now you all look like nice people. You’ve, most of you haven’t
walked out or fallen asleep, which is what I predicted. So there’s my model. It
failed. And so I’m sure you all want to save the planet. Yes. We don’t want the
planet not to be saved from global cooling. Well, when climatology was not
quite an independent field, there weren’t a lot of people doing climatology.
Back in the seventies, people really did say a new ice age was
coming. This happened, and Newsweek, 1975, I’ll read you this. There are ominous
signs that the Earth’s weather patterns have begun to change dramatically, and
that these changes may pretend a drastic decline in food production. 1974, time
climatologist, Kenneth Hare.
A former president of the Royal Meteorological Society believes
that the continuing drought gave the world a grim premonition of what might
happen. Warren’s hair, I don’t believe the world’s present population is
sustainable. We’ve doubled since then. Now there’s scores and scores of these
and scientists in groups like the un warning of mass deaths and starvation and
so forth, which did not happen.
And then we got global warming because the weather changed. It
got warm. And after a while the weather failed to continue to warm. And we got
climate change. Climate change. Now that’s a brilliant name because the Earth’s
climate never ceases changing. Not for an instant. It has never stopped
changing.
We’ll always have climate change. And so you have this weird
circular argument that the changes we’re seeing are because of climate change.
That’s what the climate does. It changes. We can’t have changes because of
climate change. So you get this, but that it’s okay, we can live with that kind
of circularity.
But that was quickly married to scientism. We’ll discuss
scientifics in a minute. When when, when doubts about climate change became
synonymous with doubting solutions to climate change, solutions to climate
change, and because of this era, the doubt you expressed in the solutions to
climate change, you’re called a climate change denier.
Now that’s an asinine name because no working scientist at all.
None. Not one denies that the climate changes or the is unaffected by man. So
we have things like this. Janet Yellen, I think it was just a week before last
at congressional Hearing, she said Climate change inflation is transitory.
Janet Yellen, that one. Janet Yellen recently said, climate
change is an existential threat and the world will become uninhabitable if, can
you see it coming if we don’t act now? Uninhabitable. Uninhabitable. That’s a
mighty word. That’s a mighty word. So these two guys were curious about this in
2021, and they looked at these apocalyptic predictions, that’s their word,
apocalyptic predictions.
And they really began around the year 1900 or so, if you can
believe it, but they didn’t pick up steam until the seventies. And the average
age of the time we have left until the end comes, there’s some variation, but
the average age is nine years. So starting with people like Paul Ehrlich in, in
the seventies, we have nine years left and we have a lot of nine years left.
We currently still have. About nine years left. The funny thing
about this, so this is what I wanna say because we’re up here preaching,
predictive the predictive method in, in science, which is necessary, but it’s
not going to save science from everything because these failures and these
apocalyptic predictions have not dented the theory or the belief in the theory
at all.
So it, it’s just predictional. It’s not gonna save us from
these kinds of a thing. All right. So I owe it to you to talk a little bit
about the science of climate change. I, we could talk about the thermodynamics
of fluid flow on a rotating sphere and all this kind of a stuff, but that’s too
much for us.
So I want to talk about the things that are said to go bad
because of climate change. What’s gonna go bad because of climate change?
Everybody know everything, absolutely everything. If it’s, if, if, absolutely.
There’s a guy in England, he, he collects what’s called a warm list. It’s a,
the site is called Numbers Watch.
He stopped collecting these a few years back. I guess he’s
elderly, I think. And he, he was up to like 900 papers and he looked at
academic papers. Here’s what’s going to happen because of climate change.
Here’s what’s going to happen. I’m just gonna read you a couple of them. These
are the, the things that are going to go bad or get worse aids.
Afghan Poppy’s destroyed African Holocaust agent deaths,
poppy’s, more potent African, devastated African conflict, African aid threat.
African aid threatened aggressive weeds. Air France crash, air pockets, air
pressure changes. Airport farewells, virtual, they nailed that one. Airport.
Malaria. Alaskan towns slowly destroyed Al-Qaeda and Taliban being helped.
Allergy increase allergy season longer. My favorite alligators
and the Thames, we haven’t even come close to getting out of the A’s. Look,
anything that is supposed to be good or benign or delicious or photogenic,
climate change is gonna kill right off. And if it’s bad, if it’s a weed, if
it’s harmful if it’s a poisonous spider or a snake, it, climate change is gonna
cause to flourish.
Now, there’s not one study that I know of that a slight
increase in global average temperature is gonna increase pleasant summer
afternoons. Nothing like this. It’s only bad things that a small change in
temperature can only be bad and it totally and entirely bad. It, it, it’s, it’s
sufficient proof. I think that science has gone horribly wrong.
It’s not logically impossible, but it can’t be believed that
changes can only be bad. Now, that doesn’t say how the beliefs are generated,
and I’m not gonna go into too much detail about this, but the way it works is
this, we first have a model of climate change, all right? And that’s not
certain. We don’t know what the predictions are gonna be exact, they’re not
absolutely certain.
And on top of that is usually some kind of weather model. We
need a weather model because you know, the alligators and the Thames are
affected by the weather. So we need a weather model on top of that, you guys
know how accurate weather models are. Then we need a model of the thing itself,
like poppy production and afg, you know, African aid and all that kind of a
stuff.
That model is even more uncertain. And finally we have a
solutions model where you say, if we do these solutions, we’re gonna be able to
fix all this kind of a stuff. Now really what you have to do is multiply these
uncertainties. It’s like multiplying a ahead after a head, after a head, after
a head on a coin flip.
It’s a half the first time, but by the time you get down to
four, it’s one 32nd, you’re just going smaller and smaller and smaller. So it’s
the bandwagon effect. It’s, it’s people jumping on these things because
everybody else is doing it. It’s just, if you’re basing it on P-values and the
like, it’s just far too easy to do and they’re all surmises.
They’re all guesses of what things are going to be like and
they’re taken as gospel. All these kinds of things, it doesn’t make any sense.
That’s it. That’s the multiplying uncertainties scientism. Scientism. I’m only
gonna talk about very little bit. Greg mentioned a little bit about it.
Scientism is the belief, the false belief. I think that science
has all the answers, not just some, but all the answers that science knows
right from wrong, good, from bad moral immor, that science knows what to do.
Well, that’s false. Science can give you predictions and give you the
uncertainty around those predictions, but that doesn’t tell you what’s right or
wrong to do.
And so that idea that when people say, we need to, this is my
program, my solution, we need to follow the science. When they say we need to
follow the science, they mean we need to follow me, because that’s what it
really comes down to. That’s not what science says. Science is absolutely mute
on right from wrong.
And there’s two kinds of science scientism I’m only gonna
bother you with one, just a real quick one. I, I call it scientism of the first
kind. Scientism of the first kind is when when knowledge that’s been obvious or
known from the farthest reaches of history is announced. Finally, as proved by
science, it’s kind of a gateway drug to the deeper kind of a science scientism,
because we don’t need scientist science to tell us what we already know.
But if we’re looking to science to tell us before we really
commit ourselves, we’re in a kind of a world of hurt. And I’ll give you one
example. The army hired a certain corporation. The U United States Army hired a
certain corporation to investigate whether there were sex differences and
physical capabilities.
Guess what they found? That’s scientism of the first kind.
Yeah, that some of it’s political cover and all that kind of stuff. I
understand, but that’s, that’s what it comes. Okay. Funny. The last you’ll be
happy to hear the last and most difficult the, what I call the eighth deadly
sin. The deadly sin of reification mistaking models for reality.
When we come to believe our models are reality, we are
committing a very bad sin. It’s, it’s very difficult to explain this. We’re in
rugged territory because we’re getting closer to the true nature of causation,
which requires a clear understanding of metaphysics and therefore the subtler
mistakes we could made in explaining it and understanding it and so forth.
And the more difficult it is to describe. Plus, I’ve kind of
taken up enough of your time, so I’m gonna give you one instance of the dead
deadly sin and two flavors, both very current. Now, I want you to just think of
this. I want you to agree with me on something here, and if we can agree with
this, we can go for it.
I think it’s an obvious fallacy to say that Y, we’re back to
our X and our Y here to say that Y cannot or has not been observed when Y has
indeed been observed because a certain X, some theory says that Y cannot be
observed. Does that make sense to you? Did I explain it? Well, we actually see
a Y. We observed this Y happening, but a certain theory, a beloved, well,
beloved theory says we can’t see the Y, therefore, what we’ve seen is not true.
I think that’s a fallacy. That’s the fallacy of the deadly sin
of reification, cuz this happens in, in, in all the time. As a matter of fact,
it’s usually when x is some cherished theory. When y is an observation that’s
scoffed at, or dismissed or explained away because it does not accord with the
theory.
The theory becomes more important than the observation. Now
this happens in the least sciences. We, we discussed some of these earlier,
like dowsing and astrology, where it’s practitioners routinely explain away
their errors. They don’t accept their failed predictions. They say no, the,
the, the causes weren’t right.
The moon was in the wrong phase. All this kind of stuff to give
all kind of reasons. But it also happens with great and persistent frequency
and the greatest of science like physics. Now, the most infamous example of our
why is free will. There’s lots of subtleties in the definition of free will,
and we can go on and on about that.
But for us, any common usage or definition will do. Well, we
all observe. We have free will. Choices confront us. We make them. There’s our
free will, there’s our why, but they say, some people say because of a certain
X, the theory of determinism, which says all there is are just blind particles
or whatever.
They are bumping into each other, following something
mysteriously called laws, explains everything. So everything is predetermined
by these things. So free will doesn’t exist. So determinism really does prove
that free will does not exist, but free will does exist because we have it. So
there must be something wrong with the theory, but they will not give up on the
theory because the theory is too beneficial for them.
Now, I promise you, you’re gonna see this this is a little
homework exercise for you. Go and look at any article out there. You’ll find
that some computer scientist or physicist or anybody explaining that free will
does not exist. And in every one of theses, you’re gonna find a variant of this
sentence, which I think is profound and hilarious.
I promise you, you’ll see this. They all say something like
this. They first say that you don’t have free will. And it’s obvious you don’t
have free will. Of course, we don’t have free will, and if only we can convince
people that they cannot make choices, they will make better choices. Look it
up, don’t trust me.
Look it up. Now, this deadly sin reification also leads to the
current mini panic over artificial intelligence ai. You’ve heard this which is
no kind of intelligence at all. All models of any kind. Only say what they’re
told to say. And AI is just a model. AI is nothing more than an abacus.
Everybody knows what an abacus is, which does calculations in
wooden beads at the direction of a superior, real, genuine intelligence. But AI
does the same thing in a computer with electric potential differences. That’s
it. There’s no intelligence there. It’s just a model doing what it’s told to
do.
There’s no reason to panic over ai. We should panic about the intelligences
behind artificial intelligence. That’s true. That’s absolutely true. Who direct
these things and use them in all kinds of ways. That’s all true. But the, the
allure and the love of theory is just too strong. They, they believe that
somehow intelligence is going to emerge just as it would’ve I guess, if you
threw a pile of abacuses over the floor over there and it’s gonna come alive
and, and become intelligent and take over the world, it just doesn’t work that
way.
And. You, you hear about emergence everywhere from quantum
mechanics on up. I always say it’s a great grand bluff. They have no proof of
it. It’s raw assertion. It’s a power play. They’ll look at you, but we’re, we
know it’s going to happen. It hasn’t happened. They, they don’t know how to
explain it. There is no theory there, but they’ll try to bluff and bully you
into believing it.
You’ll get this every time for these people. All right. There
are alternatives to this philosophy. We have no time to talk about this, like
Aristotle unionism a return to that which would do wonders for quantum
mechanics if it were better known. And the deadly center reification is much
worse than I’ve made it sound.
Much, much worse than I’ve made it sound. It leads to strange
and untestable creations like many worlds in the multiverse and things like
this in physics and gender theory, the love of model, and the love of equality
and all these kinds of theories. Well, that’s all I have to say about bad
science. Maybe I’m wrong, so I’ll leave you with the most quoted words In all
of science, more research is needed.
Thank you.
Brett Waite: We could
do a brief q and a.
Crowd: So, professor
Briggs?
William Briggs: Yes,
sir.
Crowd: In the spirit
of scientific inquiry, I may offer two points, which may be opposite of what
you were saying.
First of all, that question about immigration, it is such a
loaded and inaccurate question.
Because when I am asked that question, I’m thinking, are we
talking about legal or illegal immigration?
And whether I’m thinking about legal or illegal, I may have
different answers. So that’s number one. Okay, number two, you stated that
masks were proved to be useless, but I would beg to differ in this respect.
There are studies that were saying that the reason for
infections is that people in the grocery store walk into somebody’s sneeze
cloud, and and that’s how you get infected. It stays in the air for a certain
amount of time. Perhaps masks in that situation prevented those clouds from
forming. And I would say it would be a good thing that I can you know, thank
you that I can think of.
William Briggs: Thank
you. All right. So for the first one about immigration, excellent point. They
did in fact specify it more clearly than I’m leading out here. I’m not giving
you the whole paper, but that’s exactly right. That’s part, when you’re
investigating something like that, those ambiguities always exist in the
questions that the investigators are doing.
Absolutely. That’s part of the problem. We’re not defining
terms. They’re not rigorous and rigid enough. And I will say that it’s been
known forever that. You know, someone’s sneezing on you with a cold, you’re
going to catch a cold unless you’ve already had it and been immune. But the
problem with the masks is they just, it’s plausible.
That’s the thing. It’s plausible. My God, it must be blocking
something. I can’t breathe so well through this thing, so it must be blocking
something. But the virons, the particles themselves are so small, they just
breeze right through it. And they’ve done, there’s just dozens of, there was a
recent Cochran review that came out, Oh, just maybe a month ago or something
like this, looking at dozens and dozens of these studies and over the years,
over, over the last 50, 60 years, they just don’t work.
I mean, it’s, it would be nice to believe that they did, but
they don’t work. And, and in fact, what they do is apart from some of the
obvious things like perhaps you’re breathing bad air and all that kind of
stuff, I don’t know how important that kind of stuff is, but they do one
terrible thing. They spread fear.
They spread terrible fear. Here I am walking down the streets
of New York City. My wife and I are walking down the streets, New York City and
I’m without a mask. And here’s a guy who sees us coming. He runs off into the
street of Manhattan Street to get away from us because I must have the disease
cuz I don’t have a mask and therefore he’s gonna catch it and die.
So he runs in the Manhattan traffic. What’s deadlier? No, I
don’t, I I don’t think masks should be encouraged.
Crowd: Yeah. So you
said something that seemed to imply that it was sort of buffoonish to look for
new particles in physics.
William Briggs: No,
no, no. I don’t.
Crowd: So well, so,
you know, standard model of particle physics, you know, proposed mid 20th
century led to 30 fundamental particles all the way up to the Higgs boon a
decade ago.
And it was a measurably successful, people proposed a lot of
particles. So it seems to me that’s the next smart step, you know, to look, to
propose new particles. Of course some of them, you know, will be discovered,
others won’t, you know, I mean, that’s, that’s how science works. You toss out
different hypotheses, you see which ones will work.
So, you know, and I, I
have, I have colleagues. Here at Hillsdale and other places who, who propose
these sort of particles. So why, you know, why is that not a good idea given
the, the very high success of the standard model?
William Briggs: The
standard model has been in place since like right, 1975 or so, and the Higgs
boons relatively new, just a couple years old.
The confirmation of it. So all of these new particles that
have, these are just the past few years, they’re coming up with new particle.
They, they notice these statistical anomalies and they rush to describe them
with new, with new particle creations and new physics. They want to be the
first to explain all this kind of a stuff.
There’s nothing in inherently wrong with doing that per se,
but, There’s just lists and lists and lists of them, and none of them are
right. And why not? Instead look at look at what’s going on with the, we, we
don’t have time to discuss quantum mechanics and so forth. But that, that’s
what really where this discussion would lead.
Why not look at a different metaphysics of, of quantum
mechanics, which is out there and available, and which leads leads a different
direction than these kinds of a things. And it’s a tremendous problem because
there’s the, the, just for resources alone, all of these particles that are,
that are positive and in search for and rush to, into print, it just leads to a
lot of effort because then they have to go back and check and all that kind of
a thing.
It’s a tremendous amount of work and resources. And you think
that’s what science is supposed to be for? Okay, but the success rate’s very
low. Incredibly low. Maybe that’s good. Maybe that’s bad. Should we be paying
for it? Should all of us be paying for it? I don’t know. Don’t, that’s the kind
of questions we lead into.
I’m not saying it’s wrong. I’m saying physicists did the best
job because they went back and at least checked.
Crowd: Well, I think
there’s a couple of people that wanna follow up on that, but my topic is a
little bit different, and that is to do with the the carbon dioxide in the
atmosphere. It’s going up by five parts per million or so every year. And and,
and yet it’s never used as a measure of success.
It’s the one thing that can be measured pretty accurately, both
in the northern and the southern hemisphere. But yet nobody ever talks about
how we’re going to either slow down that increase or stop it or reverse it.
They always say it’s associated with a temperature that we’re gonna change,
which is almost impossible to measure globally.
William Briggs: Quite
right. So I’ll, I’ll handle the second part of the question, right? The global
average temperature here about is a model. It’s based on a very complex model
with gross uncertainties in it. And what they should be saying at the end, the
temperature is x plus or minus a predictive interval. And it gets into the real
weeds here when instead they talk about a parametric interval, meaning they
just talk about the model itself as if the model were true.
And that vast over certainty results about slowing carbon
dioxide down. Well, that don’t, that’s only if carbon dioxide’s a bad thing. Is
it? I mean, plants thrive, it’s plant food. I mean the, the, the, the EPA
classed it or tried to class it as a pollutant, but without it, all plants die
and then we die.
You can’t have plants without carbon dioxide. That’s what they
eat, that and water. So it’s not necessarily we, it is not, it’s not a given
that we should indeed try to reduce carbon dioxide. It’s just not a given
because. And this is well known. I don’t wanna get too much into the physics of
all this kind of a thing, but the effect carbon dioxide has on the lower
atmosphere is logarithmic, meaning it tops out pretty quickly the effect that
you build up enough CO2 and then you get some warming for that, but then you
don’t get much or any after that.
And so the only way we can have more warming is to build in
certain feedbacks. We need to build in certain feedbacks about clouds and stuff
like this, which, which are now just grossly parameterized in models that we
don’t know a lot about. And I’ll tell you about the predictive success about
climate models.
It’s not that good probably because an independent test of
climate models has never been made. You can’t have the same person who does
your books do the audit, right? That doesn’t happen. So we need to have these
models independently checked. I don’t, I, I don’t have to be on this committee,
but other people who have no nothing to gain, no interest.
And what the results are going to be. Need to check that thing.
I, I, I think such a thing is impossible because of the political climate. It
would be almost impossible for a committee doing that to come to any
conclusion. But the four ordained one, I think so we’ve seen prediction or
after prediction fail, there’s gonna be more hurricanes.
No, we have lower, fewer. They’re gonna be more intense. No,
they’re not. They’re lower, fewer. So they keep changing the metric. Instead of
looking at just strength of hurricanes, we’re gonna look at wind speed cube.
There’s good reasons to do that, and we’re gonna look at it only at certain
times in the storms.
So they keep trying to finagle and look at things that are
gonna confirm their belief. Nobody in these fields answers the one important
question. All scientists should answer. What would convince me? What evidence
would convince me that I was wrong? You ask that to people and they say, oh,
nothing. Nothing.
It’s impossible that they refuse to answer. You know, you’re
not dealing with good science there. You’re, something has gone wrong. And
that’s a very common thing in many fields. Question. Yeah. I keep looking at
this like I’m speaking into it. That’s a bad model.
Brett Waite: Dr.
Briggs, thank you so much for your speech tonight.
I want to ask about the fundamental presuppositions that guide
modern science. One of them is the idea that the universe itself is explicable
and that if we simply fund enough research will eventually have a working model
of nature in itself. But that seems to be fundamentally questionable. And it
seems that science must be fundamentally, fundamentally rooted in skepticism.
But you have a constant human inclination toward consensus and
toward a desire for honor and money and fame. And that fundamentally seems to
be the problem with modern science. And I, I wanna go back to the question I
asked. Mr. Glassman about the nature of authority versus the desire to know
That seems to be there’s a problem there that seems to need to be explored, and
I was just wondering if you could comment on that.
William Briggs:
Absolutely. That the pro, all of those things stem, you know, we all know the
answer to that question. I don’t, I think it’s it’s the obvious answer, but how
do we get ourselves out of that? How do we escape from that kind of a thing?
The problem is there’s too, like I said, there’s too much money in science,
there’s too much science going on.
The consensus just builds and builds itself cuz it keeps
growing. The number of sciences, the number of scientists keep growing and so
forth. So it looks like the, the people are becoming sure and sure when no new
evidence is being given. So the, the only thing is to try to be independent
from that.
That’s the only thing we can do. And to demonstrate that our
models are better predictive success than the other guy’s models. That’s all,
all we can, as far as the, the, the fundamental nature of, of, and the
philosophy of science goes and so forth, that’s very difficult and we need to
talk about that.
In depth. Not here. We don’t have the place for it here, but
there’s, there’s the idea that I, I don’t mean in the, in the sense that Greg
meant laws, but there’s another view of laws that people use as if these laws
are true. And these are the governing things in the world. Well, there’s
another way to think about this.
It’s not the, the laws of nature, but it’s the laws of natures.
We don’t have laws of nature. We have natures of things and things behaving
substances, behaving in ways they have powers, causal powers and things like
this. And, and typically physicists call those phenomenological laws and things
and and so forth.
And they don’t believe those are the fundamental ones, but I
think they are the fundamental ones. This is a whole. A group of philosophers
led by Nancy Cartwright and others and things like this. They, they call the,
the, the guys who think that the laws of the, the, the laws of the universe, of
the things that are guiding everything.
They call themselves the realist, which is a great name, but
but we, the other people in our camp, we have to call ourselves anti realists.
So that getting there first, computer scientists have learned that lesson.
They’re the best marketers in the world. Oh boy. Deep learning, neural nets,
machine learning, all this kind of stuff like artificial intelligence.
All completely wrong, but lovely names. Well, thank you very
much everybody.
I am a wholly independent writer, statistician, scientist and consultant. Previously a Professor at the Cornell Medical School, a Statistician at DoubleClick in its infancy, a Meteorologist with the National Weather Service, and an Electronic Cryptologist with the US Air Force (the only title I ever cared for was Staff Sergeant Briggs).
My PhD is in Mathematical Statistics: I am now an Uncertainty Philosopher, Epistemologist, Probability Puzzler, and Unmasker of Over-Certainty. My MS is in Atmospheric Physics, and Bachelors is in Meteorology & Math.
Author of Uncertainty: The Soul of Modeling, Probability & Statistics, a book which calls for a complete and fundamental change in the philosophy and practice of probability & statistics; author of two other books and dozens of works in fields of statistics, medicine, philosophy, meteorology and climatology, solar physics, and energy use appearing in both professional and popular outlets. Full CV (pdf updated rarely).
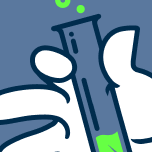
Support the Broken Science Initiative.
Subscribe today →
recent posts
In 2015 WHO said yes, but insiders say the process was flawed and biased
Where’s the Controversy? The Science of Optimum Health is largely Settled