Summary
A p value calculates the likelihood of a set of data if the null hypothesis is true: P(F|H0). People assume there is a high correlation between the probability of the findings given the null hypothesis and the reverse: the probability of the hypothesis given the findings: P(H0|F). This 2009 paper by David Trafimow and Stephen Rice is the first to test whether or not this assumption is actually true. Their findings suggest that the correlation is “unimpressive” and offers no justification for the use of p values.
The null hypothesis significance testing procedure (NHSTP) has generated a lot of controversy in recent years. This widely used procedure involves:
Defining a null hypothesis and an alternative hypothesis.
Collecting data to test these hypotheses.
Calculating a p value.
A p value is the probability of the observed data, or something more extreme, if the null hypothesis is true. A low value (less than 0.05) conventionally means that a researcher can reject the null hypothesis in favor of the alternative hypothesis.
The strongest argument against the p value is that it is logically invalid. If a rare finding has been obtained under the assumption that the null hypothesis is true, this does not conclusively prove that the null hypothesis is false. In other words a small p value, P(F|H0), does not entail a small probability of the hypothesis given the findings, P(H0|F). To get that, P(H0|F), you need to know the prior probability of the hypothesis. This involves using Bayes theorem.
To reject a null hypothesis on the basis of a small p value requires an additional assumption: that there is a correlation between the probability of data given the null hypothesis and the probability of the null hypothesis given the data. The authors set out to test whether there is a strong correlation here or not.
To run this test, the authors needed to know three key values: the probability of the data given the null hypothesis is true, P(F|H0), the prior probability of the hypothesis, P(H0), and the probability of the data if the null hypothesis is not true, P(H0|F). The first of these is simply the p value and can be determined directly from the experiment. The second is trickier. In most cases, researchers have to make subjective assumptions to determine a prior probability. And in the third, there are nearly infinite ways a hypothesis can be false. Nonetheless, the authors were able to model the effects of making various assumptions and see how they affect the results.
They calculated all three key values for 65,000 randomly generated data sets. The analysis found a very weak correlation (r = .410) between the probability of the data given the null hypothesis, P(F|H0), and the probability of the null hypothesis given the data, P(H0|F). The probability threshold for this analysis was set at .05 for each value.
The result of this analysis is that the p value fails to account for most (84%) of the variance in the probability of the null hypothesis given the data, P(H0|F). Therefore, the supposed correlation between the p value and P(H0|F) is not a “compelling justification for the routine use of p values in social science research.”
They consider a few different suggestions. One is replacing p values with a calculation of the probability of replication, prep. This calculates the probability that a second experiment with identical conditions to the first one will find results going in the same direction as the first experiment. The authors criticize the prep because it fails to consider prior probability distributions. And they have done an analysis of the prep similar to the above analysis of p values and the results were disappointing. It is unclear whether this test even measures the probability of a replication.
Another alternative to p values is Bayes theorem. This would give psychologists what they're looking for: the probability of the null hypothesis given their findings, P(H0|F). However, the authors see three potential problems: (1) no agreed upon way to determine prior probability of the null hypothesis, P(H0); (2) it is unclear how to determine the probability of the finding given the null hypothesis is not true, P(F|~H0), since a hypothesis can be false in nearly infinite ways; (3) Bayes theorem interprets probability as a degree of rational belief rather than an expected frequency. This results in an assortment of unresolved complications.
One author of this paper, Trafimow proposed an alternative method based on epistemic ratios. It is described briefly in this paper. It has a “Bayesian flavor”, but is compatible with frequentist interpretations of probability. It involves comparing two competing hypotheses. Determining the probability of the findings given either of the competing hypotheses is easily determined. The epistemic ratio of the hypotheses can then be compared to help a researcher decide which hypothesis is more favorable. Its disadvantages are that it requires researchers to devise multiple hypotheses and does not calculate the probability of any hypothesis given the findings.
The authors conclude that many previous analyses of the NHSTP did not get to the heart of the issue. Proponents have often asserted a correlation between the probability of the data given the null hypothesis, the p value, and the probability of the null hypothesis given the data, P(H0|F). But they never provided any evidence that the correlation actually exists. This paper put the hoped for correlation to the test and it failed.
P values are used by scientists to test their hypotheses, which are ideas about how something in the world might work. A p value measures how likely the results of an experiment are if the null hypothesis is true. The null hypothesis means that whatever you did in your experiment had no effect. A low p value means the result of an experiment was unlikely if the null hypothesis is true. Many people assume a low p value also means the null hypothesis is false. But no one really tested whether this was true or not till now.
The authors of this paper did the test and found that p values and the likelihood of the null hypothesis are not closely related. Finding a small p value does not mean the null hypothesis is wrong.
The authors also considered a few alternatives to the p value:
Figuring out how likely an experiment is to show the same result if you do it again
Using something called Bayes Theorem that figures out the likelihood of a null hypothesis. It involves figuring out how likely the null hypothesis was before the experiment.
Another method where you compare two different hypotheses to see which explains the results of an experiment better
Each of these methods are good in some ways, but have some downsides, too. The authors of this paper argue that there is no good reason to use p values. They have shown that one of the best reasons to use p values was actually wrong.
--------- Original ---------
ABSTRACT. Some supporters of the null hypothesis significance testing procedure recognize that the logic on which it depends is invalid because it only produces the probability of the data if given the null hypothesis and not the probability of the null hypothesis if given the data (e.g., J. Krueger, 2001). However, the supporters argue that the procedure is good enough because they believe that the probability of the data if given the null hypothesis correlates with the probability of the null hypothesis if given the data. The present authors’ main goal was to test the size of the alleged correlation. To date, no other researchers have done so. The present findings indicate that the correlation is unimpressive and fails to provide a compelling justification for computing p values. Furthermore, as the significance rule becomes more stringent (e.g., .01, .001), the correlation decreases.P values are commonly used in research to test hypotheses, which are predictions about how something works. A p value measures how likely the results of your experiment are if there is no effect of your experiment (the null hypothesis is true). Many assume a low p value also means the null hypothesis is unlikely to be true. But this assumption hasn't really been tested.
This paper tested that assumption. They found the correlation between p values and the probability of the null hypothesis being true is actually quite weak. So p values don't tell us as much about the likelihood of the null hypothesis as people assume.
The authors considered some alternatives to p values:
Calculating the probability an experiment will replicate
Using Bayes theorem to directly calculate the probability of the null hypothesis being true, which involves factoring how likely the null hypothesis was before the experiment
Comparing hypotheses using "epistemic ratios"
There are points in favor of each of these ideas, but downsides as well. The authors argue the routine use of p values in research is not well justified. This paper has invalidated an important argument in favor of p values, which is that a p value implies knowledge about the truth of a null hypothesis.
Homeschool:
Title: Understanding and Troubleshooting HTTP Error Codes: A Home-Based Learning Module
Objective: Equip parents with knowledge on internet troubleshooting, specifically on HTTP Error Codes, to support their children's homeschooling.
Tools Required: Internet-enabled device (computer/laptop/tablet), Online materials and resources, Simulation tools (optional)
Curriculum Guide:
Week 1:
Introduction and Basics of Internet Surfing
- Understanding the World Wide Web
- How Search Engines Work
- Understanding URLs
Week 2:
Introduction to HTTP
- What is HTTP?
- The Role of HTTP in Internet Surfing
- Common HTTP Methods
Week 3:
Understanding HTTP Status Codes
- What are HTTP Status Codes?
- Classes of HTTP Status Codes
- Common HTTP Status Codes and their Meanings
Week 4:
Deep Dive into HTTP Error 403: Forbidden
- Explaining HTTP Error Code 403: Forbidden
- Causes of HTTP 403 Errors
- How to Resolve Server-Side 403 Errors
- Hands-on Exercise: Identifying and Reacting to 403 error
Week 5:
Troubleshooting Guide for HTTP Errors
- General Troubleshooting Tips for HTTP Errors
- Specific Solutions for HTTP 403 Errors
- How to Seek Help when Experiencing Internet Errors
- Final Activity: Creating a Troubleshooting Guide
The above curriculum guide is subject to adjustments as parents might have varying levels of prior knowledge and tech-savviness. The pacing of the lessons can also be tailored depending on the learner's retention and understanding.
Resources are also freely available from a range of online platforms that cover both the theory and practical elements of dealing with HTTP errors. Ultimately, the goal is to empower parents with the ability to troubleshoot basic internet errors which their children might face during homeschooling.
--------- Original ---------
ABSTRACT. Some supporters of the null hypothesis significance testing procedure recognize that the logic on which it depends is invalid because it only produces the probability of the data if given the null hypothesis and not the probability of the null hypothesis if given the data (e.g., J. Krueger, 2001). However, the supporters argue that the procedure is good enough because they believe that the probability of the data if given the null hypothesis correlates with the probability of the null hypothesis if given the data. The present authors’ main goal was to test the size of the alleged correlation. To date, no other researchers have done so. The present findings indicate that the correlation is unimpressive and fails to provide a compelling justification for computing p values. Furthermore, as the significance rule becomes more stringent (e.g., .01, .001), the correlation decreases.Let's start with the truth!
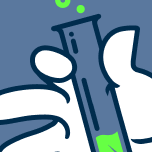
Support the Broken Science Initiative.
Subscribe today →
recent posts
Metabolic Flexibility to Burn Fat, Get Stronger, and Get Healthier
Expanding Horizons: Physical and Mental Rehabilitation for Juveniles in Ohio