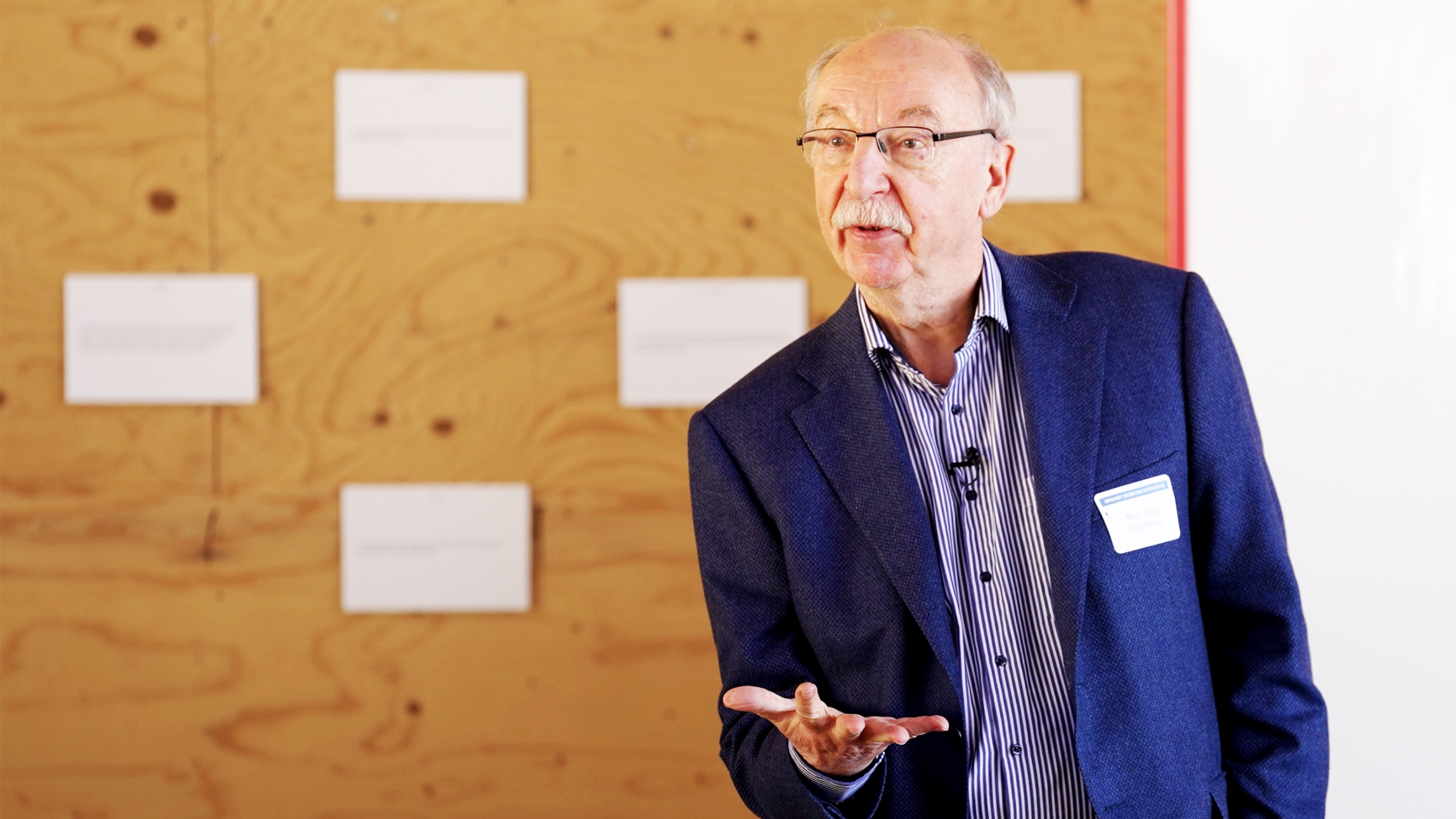
Mindless Statistics
bSI Epistemology Camp: 2024
At his talk at the Broken Science Epistemology Camp in March 2024, psychologist Gerd Gigerenzer addresses what he calls ‘mindless statistics.’ Rather than think critically about the implications, impact, and meaning of studies, researchers are stuck in a system which rewards publication and statistical significance. The pressure to publish has encouraged them to p-hack, slice data, and essentially cheat their way into showing their research is important.
Professor Gigerenzer dives into the history of statistical analysis in his field of psychology, which has led to a paradigm of rituals, in place of meaningful thought.
Professor Gigerenzer is director emeritus of the Center for Adaptive Behavior and Cognition at the Max Planck Institute for Human Development in Berlin.
Transcript
Today I will talk about a strikingly persistent phenomenon in the social and biomedical sciences; mindless statistics.
Let me begin with a story. Herbert Simon is the only person who has received both the Nobel prize in economics, and the Turing award in computer science. The two highest distinctions in both disciplines. Shortly before he died Herb sent me a letter in which he mentioned what has frustrated him almost more than anything else during his scientific career. Significance testing. Now he wrote “the frustration does not lie in the statistical test themselves, but in the stubbornness with which social scientists hold a misapplication that is consistently denounced by professional statisticians.
Herbert Simon was not alone. The mathematician R. Duncan Luce spoke of mindless hypothesis testing in lieu of doing good science. The experimental psychologist Edwin Boring spoke of a meaningless ordeal of pedantic calculations. And Paul Meehl, the clinical psychologist and former president of the American Psychological Society, called significance testing “one of the worst things that have ever happened to psychology.”
What is going on? Why these emotions? What could be wrong with what most psychologists, social scientists, and biomedical scientists are doing?
In this talk I will explain what is going wrong. The institutionalization of a statistical ritual instead of goods statistics. I will explain what the ritual is. I will explain how it fuels the replication crisis, how it brings blind spots in the mind of the researchers. And also how it creates a conflict for researchers, young and old, between doing good science, and doing everything to get a significant result.
Let me give an example. A few years ago I gave a lecture on scientific method and also on the importance of trust and honesty in science. After I finished, in the discussion section, a student from an ivy league university stood up and told me “You can afford to follow the rules of science. I can’t. I have to publish and get a job. My own advisor tells me to do anything to get a significant result.”
That’s known as ‘slicing and dicing data’ or also ‘P-hacking’.
The student is not to blame. He was honest. But he has to go through a ritual that is not in the service of science.
So let me start with the replication crisis. So every couple of weeks the media proclaims the discovery of a new tumor marker that promises personalized diagnostic or even treatment of cancer. And medical research, tumor research, is even more productive. Every day four to five studies report at least one significant new marker. Nevertheless, despite this mass of results, few have been replicated and even fewer have been put into clinical practice.
When a team of 100 scientists at the bio tech company, Amgen, tried to replicate the findings of 53 landmark studies, they succeeded only with six. When the pharmaceutical company, Bayer, examined 67 projects on oncology, women’s health, and cardiovascular medicine, they were able to replicate only 14.
So what do you do when your doctor prescribes you a drug based on randomized trials that showed that’s efficient, but then it seems to fade away. Now, medical research seems to be preoccupied by producing non-reproducible results.
Ian Chalmers, one of the founders of the Cochrane Society, and Paul Glasziou, chair of the International Society for evidence-based Health Care, estimated that 85% of medical research is avoidably wasted. And they estimated a loss of $170 billion every year worldwide.
The discovery that too many scientific results appear to be false alarms has been baptized the ‘Replication Crisis’.
In recent years a number of researchers, often young researchers, have tried to systematically find out to what degree the problem is. And typically the results show that between 1/3 and 2/3 of published findings cannot be replicated. And among those who can be replicated the effect size is on average, half.
So in medical research for instance the efficacy of anti-depressants plummeted drastically from study to study. And second generation anti-psychotics that earned Eli Lily a fortune, seem to lose their efficacy when retested.
It’s interesting how the scientific community reacted. So what would you do if your result that made you famous, disappears? Some researchers like the psychologist Jonathan Schooler faced the problem and tried to think about what’s the reason. And Jonathan came up with the idea of ‘cosmic habituation’. In his words it was as if nature gave me this great result and then tried to take it back.
The New Yorker called this ‘The Truth Wears Off’ phenomenon. Others reacted, so other researchers reacted differently, and we’re not happy with those who tried to replicate their studies and failed, and waged personal attacks on those speaking of, I quote, ‘replication police, shameless little bullies, which hunts,’ or compared to to the Stasi.
So here we are. At the beginning of the 21st century one of the most cited claims in the social and biomedical sciences was John Ionnidis, ‘Most Scientific Results are False.’
In 2017, just to give a hint about the possible political consequences the news website breitbart.com headlined a claim by Wharton School, Professor Scott Armstrong, that I quote “fewer than 1% of papers in scientific journals follow scientific method.’ End of quote.
Now we we have seen in this country and in other countries, politicians trying to cut down funding of research. And if they would read more about this there would be more going in this direction. And those who point out that so many results are not replicable, they face a double problem. They want to save science, at the same time they run the danger that maybe Donald Trump, someone else, will use this to cut funding totally down.
So how did we get there?
The replication crisis has been blamed on economic, on false economic incentives, like ‘publish or perish,’ and I want to make a point today that we need to go beyond the important role of external incentives and focus on an internal problem that fuels the replication crisis. And this is the factor that good scientific practice has been replaced by a statistical ritual.
My point is resources follow this ritual not because, or not only because of external pressure. No, they have internalized the ritual and many genuinely believe in it and that can be seen most clearly by the delusions they have about the P-value, the product of the ritual.
So statistic methods are not just applied to a science, they can change the entire science. So think about parapsychology, which once was to study of messages by the dear departed, and it turned into the study of repetitive card guessing. Because that’s what the statistic method demanded.
In a similar way the social sciences have been changed by the introduction of statistical inference. And typically in social science, scientists first encountered Sir Ronald Fisher’s theories, and particular his 1935 book. He wrote three books. The first was too much about agriculture and manure, and technically too difficult for most social scientists. But the second one was just right. And it didn’t smell anymore.
And so they started writing textbooks. And then I became aware of a competing Theory by the Polish statistician, Jerzy Neyman, and a British statistician, Egon Pearson.
Fisher had a theory, so at least his null hypothesis testing, where he had just one hypothesis, Neyman insisted that you need two. Fisher had the P-value computed after the experiment. Neyman and Pearson insisting everything in advance.
I’ll just give you an idea about the fundamental differences, and I give you an idea about the flavor of the controversy. Fisher branded Neyman’s theory as ‘childish’ and ‘horrifying for the freedom of the West,’ and linked Neyman-Pearson theory to Stalin’s five-year programs. Also to Americans who cannot distinguish or don’t want to distinguish between making money and doing science. Incidentally Neyman was born in Russia and moved to Berkeley, in the U.S.
So Neyman, for his part, responded to some of Fisher’s tests and said “these are in a mathematically specifiable sense, worse than useless.” What he meant with is that that the power was smaller than Alpha. Such in the famous lady T test.
So what do textbook writers do when there are two different ideas about statistical inference?
One solution would have been, you present both. And maybe also Bayes or Tukey, and others, and teach researchers to use their judgment to develop a sense in what situation it’s not working and where it’s better to do this. No, that was not what textbook writers were going for. They created a hybrid theory of statistical inference that didn’t exist and doesn’t exist in statistics proper. Taking some parts from Fisher some parts from Neyman, and adding their own parts, mostly about the idea that scientific inference must be without any judgment.
That’s what I mean mindless… automatic.
And the essence of this hybrid theory is the null ritual.
The null ritual has three steps. First set up a null hypotheses of no mean differences, or zero correlation. And most important, do not specify your own hypothesis or theory, nor its predictions.
Second step. Use 5% as a convention for for rejecting the null hypothesis. If the test is significant claim victory for your hypothesis, that you have never specified. If the test result and report the test results as P smaller than 5%, or 1%, or 0.1%, whichever level is is met by your results.
And the third step is a unique step. It says always perform this procedure. Period.
Now neither Fisher, nor to be sure, Neyman-Pearson would have approved of this procedure, and Fisher for instance said ‘no scientific researcher will ever have the same level of significance from experiment to experiment.’ He will give his thoughts. Neyman also and Pearson emphasized the role of judgment. And if the two fighting camps agreed on one thing it was scientific inference cannot be mechanical. You need you use your brain.
And that was exactly the message the null ritual did not convey. Namely it wanted a mechanical procedure. Where we can measure the quality of an article.
Now what did the poor readers of these textbooks do with a mishmash of two theories which were not mentioned that it is a mishmash, not in names of Neyman and Pearson attached to the theories. So the result was that the external conflict between the two groups of statisticians went into an internal conflict in the average researcher.
I use a Freudian analogy to make that clear. So the super ego was Neyman-Pearson theory. So the average researcher somehow believed that he or she had to have two hypothesis and and actually give thought about Alpha and the power before they experiment and calculate the number of subjects you need. But the ego, the Fisher in part, got the things done and published. But left with a feeling of guilt of having violated the rules. And at the at the bottom was the Bayesian Id, longing for probabilities of hypothesis, which neither of these two theories could deliver.
How did all this come apart? So how could this happen?
I’ll give you another story. I once visited a distinguished statistical textbook writer whose book went through many editions and whose name doesn’t matter. He was one of the only ones, actually his book was one of the best ones in the social sciences, and he was the only one who had in an early edition, a chapter on Bayes, and also, albeit only one sentence, mentioning that there is a theory of Fisher and a different one of Pearson. Neyman-Pearson.
So to mention the existence of alternative theories was unheard of, and even names attached to that. So I asked him why he took out the chapter on Bayes and this one sentence, from all further editions. When I met him he was just busy having, I think, was the fifth edition of his bestselling book. And why he, I asked him, created a inconsistent hybrid that every decent statistician would have rejected. To his credit, I should say that he also did not attempt to deny that he had produced an illusion. But he let me know whom to blame for it, and there were three culprits.
First, his fellow researchers. Then, the university administration. And third, his publisher.
His fellow researchers, he said, are not interested in doing good statistics. They want their papers published. The university administration promoted people by the number of publications, which reinforced the researchers attitude. And his publisher did not want to hear about different theories. He wanted a single recipe cookbook and forced him, so the author told me, to take out the Bayesian chapter, and even this single only one sentence about Fisher, and Neyman and Pearson series.
At the end of our conversation I asked him in what statistical theory he himself believes. And he said deep in my heart I’m a Bayesian. Now if they also was telling me the truth, he had sold his heart for multiple editions of a famous book whose message he did not believe in.
10,000 students have read this text believing that it reveals the method of science, dozens of less informed textbook writers copied his text, churning out a flood of offspring textbooks inconsistent, and not noticing the mess.
I have used the term ‘ritual’ for this procedure for the essence of the hybrid logic because it resembles to social rights. Social rights have typically the following elements. There are sacred numbers or colors. Then, there’s a repetition of the same action, again and again. And then there’s fear. Fear about being punished if you don’t repeat these actions. And finally delusions. You have to have delusions in order to conduct the ritual.
The null ritual contains all of these features.
There’s a fixation on 5%. And in functional MRI it’s colors. Second, there’s repetitive behavior resembling compulsive handwashing. And third, there’s fear of sanctions by editors or advisers. And finally, there’s a delusion about what a P-value means, and describe that in a moment.
Let me just give you a few examples about the mindless performance of the ritual. They may be funny, but deep it’s really disconcerting.
So in an internet study on implicit theories of moral courage, Philip Zimbardo, who is famous for his stain for prison experiments, and two colleagues, asked their participants “do you feel that there is a difference between altruism and heroism?”
Most felt so. 2,347 respondents said ‘yes’ and only 58 said ‘no.’ Now the authors computed a Chi-Squared test to answer whether these two numbers are the same or different. And the found that they indeed different.
A graduate student of mine, a smart one, had the opposite situation. His name is Pogo. Pogo ran an experiment with two groups and found that it two means are exactly the same but Pogo could not just write that or say that. He felt he had to do a statistical test, a T test to find out whether the two exactly same numbers differ significantly, and he found out they don’t. And the P-value was impressively high.
Here’s the third illustration. I recently reviewed an article in which the number of subjects was 57. The authors calculated a 95% confidence interval for the number of subjects and concluded the confidence interval is between 47 and 67.
Don’t ask why they did it. It’s mindless statistics.
Almost every number in this paper was scrutinized in the same way. The only numbers in the paper that had no confidence were the page numbers.
This is an extreme case but unfortunately it’s not the exception. Consider all behavioral neuropychological and medical studies in Nature in the year 2011. 89% of the studies report only P-values and nothing else that’s of importance. Such as no effect sizes. No power. No model estimates.
Or an analysis of the Academy of Management Journal, in a year later reported that the average number of P-values in an article is… guess how many P-values. If you have two hypotheses you would need two. No. 99.
Yeah it’s a mechanical testing of any number. So the idol of automatic universal inference, however is not unique to P-values, or confidence intervals. Dennis Lindley, a leading advocate of Bayesian statistics, once declared that the only good statistic is Bayesian statistics and that Bayesian methods are even more automatic, in his opinion, than Fisher’s run methods.
So the danger is here. You don’t have much progress if you use Bayes factors just as mindlessly. So let me go on. The examples about mindless use sound funny, but there are deep costs of the ritual. I’ll give you a few examples.
Maybe the first and quite interesting one is that you actually fair better if you don’t specify your own hypothesis. Why?
Paul Meehl once pointed out a methodological paradox in physics. Improvement of experimental measurement and amount of data, make it harder for a theory to pass. Because you can more easily distinguish between the prediction of the theory and the actual data. In fields that rely on the null ritual it’s the opposite.
So, because it tests a null in which you don’t believe. So improvements of measurement make it more easier to detect the difference between the data and the null, and that means more easier to reject the null and you can collect victory for your hypothesis. And you can’t just imagine that it’s another factor that leads to the irreplicability of results.
Second point and, I now get to the delusions. And about this sacred object, the P-value. Now a P-value is the probability of a result, or a more extreme one, if the null hypothesis is correct. And more technically correct, it is the probability of a test statistic given an entire model. But the point is it’s a probability of data given hypothesis, not the Bayesian probability. And that should be easy to understand for any academic researcher.
And also it’s not the probability that you will be able to replicate it. And the replication delusion, that’s the first one, is that when you have a P-value of 1%, then logically it follows that the probability you can replicate your result is 99%. Clear?
So this illusion has been already told in the book that Greg pointed out by Nunnally. It’s great reading. if you have fun, you want to really have fun, read the old textbooks about statistics. They’re all not written by statisticians otherwise they wouldn’t have been used.
So instance Nunnally writes, quote, “what does a P-value of 5% mean?” His answer: “The investigator can be confident with odds of 95 out of 100 that the observed difference will hold up in further investigations.” That’s the replicability delusion.
So I was curious, what is the state today?
Do academic researchers understand what a P-value means? So the the the object they looking for.
So I surveyed all studies available in six different countries with a total of over 800 academic psychologists, and about thousand students, and they were all asked “what does the P-value of 1% mean?” How many of these professors and students cherish the replicability illusion.
So in this example there were 115 persons who taught statistics. But you should know that in the social sciences, mostly and certainly not in psychology, those who teach statistics are not statisticians. For the same reason, because they would notice what’s going on.
So what do you think? What proportion of 115 statistics teachers, that’s across six countries, fall prey to the illusion of reputability? It should be zero. It is 20%.
Then we have looked at the professors, and that’s over 700 in this study. In the professors it’s 39% who believe in the replicability illusion. So almost double.
And among the poor students it is 66%. They have inherited the delusion.
And note that this is another reason why there replicability crisis is not being noticed until recently, because of this illusion. I have a problem, it is a P-value of 1%, I can be almost sure it can be replicated.
Now it’s not the only delusion that is shared by the academic researchers. So the next delusion is that you think that P-value is the probability of data given a hypothesis, tells you about the probability of the hypothesis given the data. And the majority of academic psychologists in six countries and in every study, shares at least one ,and typically several, of these Illusions. Including that the P-value of 1% tells you that the probability that null hypothesis is true is also 1%, or that the alternative hypothesis is true is 99%. And so it goes on.
This is a remarkable state of the art of doing science. Remarkable because everyone of these academic researchers understands the probability of A, given B, is not the probability of B, given A. But within the ritual thinking is blocked.
So another blind spot and cost is of obviously effect size. There is no effect size in the ritual. There’s an effect of in Neyman and Pearson, yeah. But that’s not being told.
McCloskey and Ziliak asked the question “do economists distinguish between significance, and so statistical significance, and economic significance?”
And I looked at the papers in one of the top journals, The American Economic Review, and of 182 papers, 70% did not make a distinction. And what Ziliak and McCloskey did, they published the names of those who got most confused, including a number of Nobel laureates. 10 years later they repeated that, assuming that everyone must have read that and people are now more reasonable, but the 70% who confused it, the number didn’t go down, it went up to 82.
Similar, there’s a blind spot for statistical power. There is no power in the null ritual. And power means the probability that you find an effect if there is one. And that should be 80%, 90%. Better higher.
The psychologist Jacob Cohen was the first one who systematically studied the power of a major clinical journal, and he found that the average power for a medium effect was 46%. That wasn’t much. Now, Peter Sedlmeier and I, 25 years later, which should be a time that things change, looked and analyzed the power in the same journal: Before it was 46, it went down to 37. Why? Because many researchers now did Alpha adjustment which decreases the power.
And notice what that means. If you if you set up an experiment that has only a power of say 30%, to detect an effect, if there’s one, you could do better and much more cheaper by throwing a coin and you would have a power of 50%. Clear?
And you could spare all this effort, and what I’ve told you now are even the better results. Studies in Neuroscience, so for instance, studies about Alzheimer’s disease, genetics, cancer biomarkers, the median power of more than 700 studies is 21%.
In F functional MRI studies only 8%. And a recent study that has looked at 368 research areas in economics and analyzed the 31 leading journals, found a median statistical power, again calculated for an alpha of 5% and a median effect size in the area, what would you guess? Economists, 7%.
Then they looked on the top five, so economics has a hierarchy, the top five. What’s there? What do you think? 7% in the hoi polloi. In the top five only 5%.
Low statistical power is another reason for failure for replication, and the interesting thing is it’s not being noticed.
There’s a recent study by Paul Smaldino and Richard McElory have looked at 60 years of power research in the behavioral sciences. They took every study that has referenced our study, there with Peter Sedlmeier. So in order to get studies, a large number of studies, and they found consistently low power and it’s not progressing. And one of the reasons is blindness in the null ritual.
Let me get to a final point about the costs. It is the moral problem. So science is based on trust and the honesty of researchers. Otherwise we can’t do this. And this statistic ritual creates a conflict between following the scientific morals, or trying to do everything to get a significant result even, if it’s a false one.
And that’s called ‘p-hacking’. That’s called ‘borderline cheating.’ Borderline cheating because you don’t really invent your data but you slice and you calculate maybe in this slicing, or in that slicing, as long you find something. And borderline cheating includes: You do not report all the studies you have run but only the one where it’s significant. You do not report all the dependent measures you have looked at but only the signal. You do not report all the independent measures, and maybe if your if your P-value ends up is 5.4%, you round it down slightly under five.
And if you analyze the the distribution of P-values, you see exactly, there is those are missing who is slightly above. And those are too high or bit lower.
So a study by John, Loewenstein, and Prelec, with over 2,000 academic psychologists found that the far majority admitted that they have done at least one of these questionable research practice that amounts to cheating. And when they were asked whether the peers do it, the numbers were even higher.
So let me go come to my end, and ask what can we do about all this?
And the simplest answer would be we need to start, or we need to foster statistical thinking. not rituals. And the crisis, there have been proposals made, The American Statistical Association has made a number of statements which I think were not very helpful.
A group of 50 researchers, all luminaries, has recommended a solution, namely to change P equal or smaller than 5% to P smaller than 0.5%. That makes it harder but it doesn’t even address the problem.
What will happen? There will be more intensive P-hacking because you have to work harder to get this. Right?
I think we have to make more fundamental changes and I can here only sketch that. First we need to finally realize that we should test our own hypothesis, not a null.
Second, we need to realize that the business is about minimizing the the real error in our measurement. Not taking the error and dividing it by the square root of N.
So this is a key disease and here’s another story. I once was a visiting scholar in Harvard and it happened that I had my room, my office next to B.F. Skinner’s office. So B.F. Skinner was once the most most well-known and controversial psychologist. And at that time he was quite old, and his store was going down because of criticism. And he felt a little bit lonely, that was my impression, so we had lots of time to talk.
And I asked him about his attitude to statistical testing. It turned out that he had obviously no recognizable training in statistics but he had a good intuition. He said he admitted that he once tried to run 24 rats at the same time. He said “it doesn’t work because you can’t leave keep them at the same level of deprivation and you increase the error.”
And he had the right intuition. It’s the same intuition as Gosset, the man who under the pseudonym, Student, developed a T-test said “a significant result by itself is useless. You need to minimize the real error.”
Skinner told me this story that when he gave an address to the American Psychological Association, and after having reported about one rat, he said “according to the new rules of the Society I will now now report on the second rat.”
So he understood that part.
And a third move, besides really taking care of what you measure, and in many physics experiments weeks and months are spent on trying to get clear measurements. In the social science it’s often Amazon Turk workers, who somehow answer questions for little money, in short time, and they do best if they don’t really pay attention.
So the third point would be remember that statistics is a toolbox. There is no single statistical inference method that is the best in every situation. One often needs to tell this to my dear Bayesian friends. Bayes is a great system, but it also doesn’t help you everywhere.
And universities should start teaching the toolbox and not a ritual.
And editors are very important in this business. They should make a distinction between research that finds a hypothesis and one that tests the hypothesis. So that young scientists don’t have to cheat anymore and pretend they would have the hypothesis that they got after the data, already before.
Second, editors should require when inferences are made to state the population to which the inference refers. People or situations. And many application of statistics there is no population. There is no random sample. Why do we do the inference, and to what population? Unclear.
Third, editors should require competitive testing and not null hypothesis testing.
And finally, in my opinion one important signal would be that editors should no longer accept a manuscript that reports results as significant or not significant. There’s no point to make this division, and it’s exactly the problem that then people try to chea,t or fail to have this.
If you want to report P-vales, fine, but report them as exact P-value. That was what Fisher in his third book, in the 1950s, always said. Fisher rejected the idea to have a criteria because that is what he meant, five year plans.
And at the end, I want to put this in a larger context. The null ritual is part of a larger structural problem that we have in the sciences. And the problem is that quality is more and more being replaced by quantity.
As Noble laureate in physics, Peter Hicks said:
“Today,” he said, “I wouldn’t get an academic job. It’s as simple as that. I don’t think I would be regarded as productive enough.”
And we have come into an understanding of science, that science means producing as many papers as possible. That means you have less idea to think. And thinking is hard. Writing is easy.
And one driver of this change from quality to quantity, are the university administrators who count rather than read, when the deciding on promotion or tenure.
A second driver is the scientific publication industry. Sorry, the scientific publishing industry, that misuses the infinite capacity of online publication for making researchers to publish more and more, in more and more special issues, and in more and more journals.
This development towards quantity instead of quality is further fueled by the so-called predatory journals that emerged in the last 20 years. Predatory journals are journals are obviously only for collecting a few thousand bucks from you, from publishing your paper with no noticeable review system. We know cases where reviewers, these are often serious scientists who somehow do not notice what’s going on, and then reject the paper, and they were told clearly that it’s not in the interest of the publishing company.
And most recent we face an new problem. Namely the industry like systematic production of fake articles with the use of AI by so-called paper mills. A paper mill, so assume you are, and it’s it’s mostly in the biomedical sciences in genetics. Assume you work in a hospital, you’re a doctor, and you need an article in a good journal to be promoted. And somehow it doesn’t work. So paper mill offers you, it’s called assistant services. Papermill offers you for 10,000 or $20,000 to write an article that’s actually faked from the beginning to the end, including maybe, western plots that are faked, and they can guarantee significance, and they can guarantee publication.
And why? Because more and more in the last years they bribe editors from journals to publish the papers they sent them, and pay them—like a colleague of mine who is an editor of medical journal, he got an offer from a paper mill in China— that for every article you publish we pay you $11,000 multiplied by your impact factor. And we will help you to increase your impact factor.
So, talking about broken science. That’s the future. So let me finish. I think the larger goals are scientific organizations like the Royal Society of London should take back control of publishing. Out of the hands of commercial pro-profit publishers. That can be done. For instance it happened last year. The top journal in neuroscience, NeuroImage, 42 editors of NeuroImage stepped back. Resigned because of the what they called ‘greed of Elsevier,’ and founded a new journal called Imaging Neuroscience. And made a call to the entire scientific community, submit your papers to the nonprofit journals and no longer support the exploitation of reviewers, of writers, of editors, by these big publishing companies who in some years make more profit than the pharmaceutic industry.
So that’s one way. And a second important conclusion is universities need to be restored as intellectual institutions, rather than run as if there were corporations.
We need to publish fewer articles and better science. We need statistical thinking. Not rituals.
Thank you for your attention.
[Applause]
Thank you so much.
Q&A
Questions?
[Haavi Morreim]
[I’ve got one quick. You’ve you’ve outlined this, the myriad of factors contributing to the replication crisis. Could I pile on by adding one more? Grant giving entities, whether government or private, aren’t interested in doing this. They paying you to do what somebody else already did. What’s already been shown. They want you to plow new ground. Show us something new. Whatever.
There’s no money in replicating. Or unless there is some specific reason to question what’s, quote unquote, “already been shown.” And I I live inside an academic Medical Center and you can publish all you want but if you didn’t bring cash, okay, grants and overhead and all that kind of thing. I love your ideas. Who’s going to pay for it. The financing system needs to be a part of the resolution.]
Yeah you’re totally right. And there are more factors. And what we can do is from below, from the ground, and stand up as scientists and use our own values to change the system. And the system had worked some time before. And we are in the danger to let it go in the hands of commerce.
[Jay Couey]
[Yeah, I thought what you said about the two different kinds of science, like whether you’re testing a hypothesis or defining it, I think Neuroscience is very much trapped in this idea that they’re testing hypothesis yet they’re still trying formulate one. And their null hypothesis, not they don’t understand. That’s the problem. I think something you can add, the null hypothesis of your assumption, or it might be this but it’s not.]
Yeah, you’re totally right. And the response to what you’re saying is often “yeah, it’s too difficult. We cannot make precise predictions.”
My response to that response is, it’s not too difficult, but the reliance of neuroscience is mostly the null ritual. That invites you not to think about theory. Real theory. And about precise hypothesis. And then you just continue this state of ignorance. But you get your papers published.
[Malcolm Kendrick]
[Can I just make one point, which is in the medical world if you do a study on say statin versus a placebo, and you prove statistical whatever, rubarb, you’re never allowed to do that study again, ever, because you proved that the drug is better than placeo. You can never have another placebo arm, ever. And I’ve been thinking about this. I don’t know what the answer is but it means that once you’ve proven your drug works you can never do any reputation or reproducing that study ever again.]
Do we getting together the pieces for Broken Science? Here’s another one.
[Peter Coles]
[Yeah, I just I mean I agree with everything you said about the confusion in academics about whether they’re talking about the probability of the data given the model, or the probability of the model given the data. So given the level of confusion that exists within the research community it’s not surprising that when it comes to the public understanding of science it’s a complete nightmare, because non-specialist journalists garble this meaning of the results even more than the academics do, probably.
So we end up with very misleading press coverage about what results actually mean— what has been discovered, what has not been discovered—and that’s really damaging for public trust in science, and also obviously, has implications for you know, kind of political influence on science as well. If they see the public trust in science disappearing, and it’s all part of this problem that scientists and the media do not communicate their ideas clearly enough for people to understand.
What I would say just to conclude is that um very, very often in science the only reasonable answer to a journalist’s question is “I don’t know,” because in many, many situations we really don’t know the answer. But you don’t get on in your career if you keep answering questions like that, even though it’s true that you don’t really know the answer. The journalists will push you into saying “oh yes I prove this is true because my P-values.” So there’s a much wider…]
One solution to this would be systematic programs to train journalists. A few of them exist. I have been participating in the, but it really needs to be on a broader basis, and it’s becoming more and more difficult because journalism is more… less investigative. But more from one day to another one.
[Peter Coles]
[Well the main media outlets are sacking journalist essentially.]
Competition through social media is tough. And at the end we may at some point face a situation where it’s no longer clear what is truth and what is fake. And we need to be prepared, and we need to prepare the public for that.
[Yeah okay. I do have a question. So you talked about the idea of we need to have fewer papers. Fewer better papers. And just looking at the idea of there’s so many papers that are not reproducible, to me suggest that there’s actual no science there. Okay. So is there enough new science to write enough papers to go around?]
Yes. The moment you stop: Predatory journals – they need papers. And also you stop publishers like Frontiers, who in between 2019 and 2021 has doubled the number of papers they put out.
So your question “are there enough papers for the pro profit publishers that we have now?” For most of them there are never enough papers.
[Yes. So I’m actually saying a slightly different thing.]
Yeah.
[If I’m a researcher are there actually enough ideas to write enough papers to like do anything useful?]
Yeah that’s a good question. Fair question. One answer would be if you have no ideas you shouldn’t be a researcher. Do something different.
And also we need to see… so university departments need to be care careful in hiring but let people have time to really develop some ideas, and take the risk. That there are few. But we don’t profit from the mass production of average things. Yeah. Yeah.
[Following up on on his question. I had a teacher in graduate school who had a prophylactic solution or contribution to this debate. He thought that instead of encouraging teachers to publish, we should discourage them, or at least we should give them an incentive to think very carefully before they published. He proposed docking them $1,000 for every article they published and $5,000 for every book.]
[Of course that was quite a while ago so you would have to increase those numbers to account for inflation. I think there’s something to be said for that.]
So students would have to pay?
[So the the teacher would have to pay. In other words it would encourage him to really think he had something to say. Because that it would be worth $1,000 to publish this article or $5,000 to publish the book. He the teacher… his salary let’s say would go down.]
[Graciano Rubio]
[Would you say that there’s value in using the P-values as a way to allocate resources for studies that deserve to be repeated and a way using those resources on new research and publishing more papers? So in in regards to the the charity and foundation for the philanthropic events, there’s always limited resources. You only have so much time and money. So if we accept that the P-value is not sufficient for validation could you use the P-value as a way to say these are the studies which deserve to be repeated. So that we can we can find results that are predictable and away from going out and trying to find something new and just focusing on the volume of papers.]
Yeah. So I understand your question there’s a conflict between replication and finding something new. Yeah. Certainly yes. But I think there are enough researchers who might focus on replication. For instance —it could be an answer to your question— those who think that at least at the moment have no new ideas… do the replication. Police. And others to find new ideas. But new ideas are hard to come by. We need to patient with them. And most of us, if you have one great idea in your life that’s already above average.
[Peter Coles]
[Am I allowed a second second one? It occurred to me a long time ago that part of the pathology of the academic system is actually the paper itself. The idea of a paper. Science has become synonymous with writing papers. Whereas if you look at the tech, the world we have now, digital publication. We don’t have to write these tiny little Quant of papers and get publications. You don’t communicate science effectively that way anymore. It’s a kind of 18th century idea that you communicate by these written papers. You could have living documents for example, which are gradually updated as you repeat. But of course the current system does not allow a graduate student to get promoted, to get advanced in that way. But I think by focusing entirely on papers we’re really corrupting the system as well. It’s not so much an issue about who publishes them, although it’s a serious one as you said. It’s the fact that we’re fixed in this mindset that we have these little articles that we have to communicate only by these articles. And that’s forcing science into boxes which is not really helpful.]
We can talk about this over lunch, but I’m still with papers. I think papers, and also books, or patents, depending on the realm, are still good. But the number of the papers is the problem. And also this edifice that you have, that all that counts is significance instead of effect size. Good theory. A good theory can predict a very small change.
[Anton Garret]
[Can I add to that that Peter Medawar, who is a Nobel prize winning immunologist, wrote an essay called “Is The Scientific Paper A Fraud?” I think several decades ago. And he didn’t mean that the results were fraudulent. What he was saying was that a scientific paper as the end product does not reflect the process by which it’s created.]
Yeah. Yeah. And that would be very helpful for students. To see the agony that goes into writing a paper the ever, ever changing of the thing. And that would help students very much, because they think “Oh, I never will achieve this.” The final product.
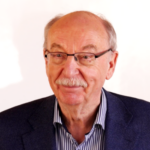