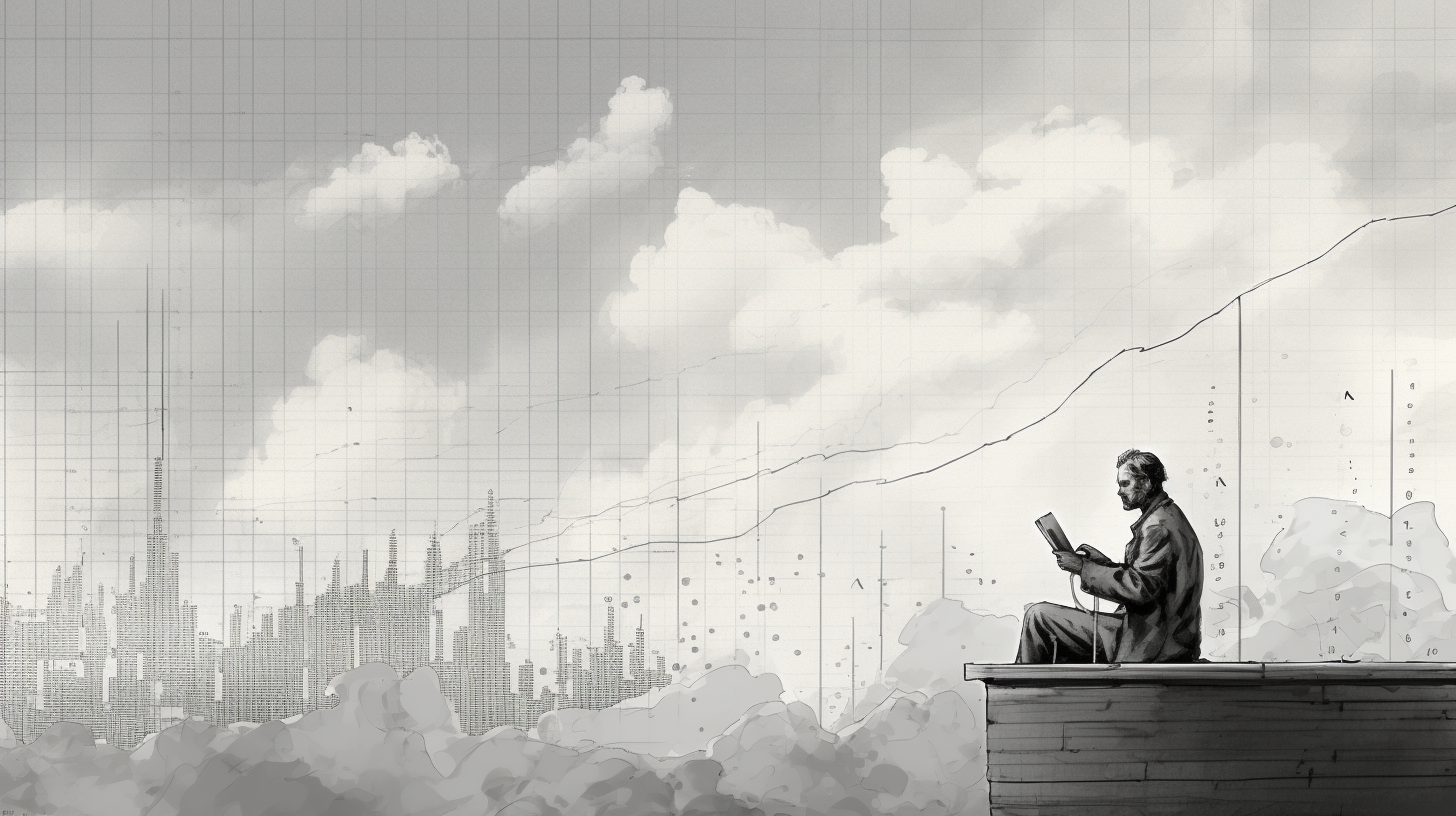
Scientific method: Statistical errors
Summary
This article by Regina Nuzzo takes a close look at the P value, considered the “gold standard of statistical validity.” Despite its widespread use, it might not be as reliable as most scientists think. She tells the story of Matt Motyl, a psychology PhD student who researched political extremists. His hypothesis was that political moderates could more accurately perceive shades of gray more accurately that left or right-wing extremists. The initial data supported the hypothesis, eliciting a P value of 0.01 which is conventionally interpreted to mean “very significant”.
To be extra cautious, Motyl and his adviser, Brian Nosek, replicated the study. However, the replication did not find a significant result. Its P value was 0.59. Motyl's “sexy” hypothesis evaporated and so did his hopes of publication. Apparently, extremists do not literally see the world in black and white.
Motyl had done nothing wrong. The P value was to blame. In 2005, epidemiologist John Ioannidis published his now-famous paper, Why Most Published Research Findings are False. It shed light on the replication crisis, which has only gotten worse since then. Scientists are now re-thinking how they go about their work, including the use of P values.
P values have been criticized by statisticians since they were introduced by Ronald Fisher in the 1920s. One sarcastic researcher suggested they be renamed “Statistical Hypothesis Inference Testing,” which makes sense once you consider its acronym. Even the inventor of the P value did not intend for it to be used the way it is now. It was meant to be a quick way to see if the results of an experiment were “consistent with random chance.” This involved a series of steps:
Define a null hypothesis, predicting “no correlation” or “no difference” between the two groups.
Assuming the null hypothesis is true, calculate the likelihood of getting similar or more extreme results compared to what were observed.
This probability is the P value. A high value means the results are consistent with the null hypothesis. A low value means the null hypothesis can be rejected.
A P value is intended to measure “whether an observed result can be attributed to chance.” But it cannot answer whether the researcher's hypothesis is true or not. That depends in part on how plausible the hypothesis was in the first place. An implausible hypothesis with a low p-value will become only slightly more plausible.
Fisher's rivals, Jerzy Neyman and Egon Pearson, promoted an alternative system based on statistical power (how likely an analysis is to detect an effect). The method could be calibrated based on the relative importance of false positives and false negatives. Notably missing was the P value. Neyman called Fisher's work, “worse than useless.” Fisher called Neyman's approach “childish” and accused the Polish mathematician of being a communist.
On the outside of this bitter battle, researchers who were not statisticians began writing manuals for working scientists that taught a hybrid approach. It combined the ideas of Fisher, Neyman, and Pearson, but would not be endorsed by either. It was procedural and rule-based like the Neyman-Pearson system and utilized Fisher's simple P value. They declared that a P value below a threshold of 0.05 was “significant”. This was never Fisher's intent, but that is how it is used today.
Most scientists are confused about what a P value really means. Most would say that a value of 0.01 means there is a 1% chance that a result is a false positive. However, this is fallacious. The P value can only describe the likelihood of the data assuming the null hypothesis is true. But it cannot say anything about the likelihood of an effect caused by the experimental hypothesis. To do this requires knowing the odds that such an effect exists. Nuzzo offers the example of waking up with a headache and thinking you have a rare brain tumor. It's possible, but still unlikely. The more implausible a hypothesis is, the more likely a finding is a false positive, even with a small P value.
Some statisticians have attempted to provide a rule-of-thumb to calculate the likelihood of a true effect given a small P value. For example, a P value of 0.01 implies a probability of at least 11% that you have a false positive. For a P value of 0.05, this probability increases to at least 29%. In the case of Motyl's study on political extremists, his P value of .01 meant there was at least an 11% chance the study would not replicate and only a 50% chance of finding another result of similarly high significance.
A major criticism of the P value is that it does not show the actual size of an effect. Nuzzo mentions a study of divorce rates to illustrate this point. People who met their spouses online were significantly (p<.002) less likely to divorce than people who met offline. However, the actual difference in divorce rates was 5.96% versus 7.67%. Just because an effect is “significant” does not mean it actually matters in the real world.
Another issue with P values is that researchers have learned ways to get around a too-high-to-publish P value (p>.05). The term for this, coined by Uri Simonsohn, is “P-hacking”. Essentially it means trying multiple things until you find a significant result. This can include peeking at data, prolonging or stopping an experiment once significance is reached, or data mining for some combination of factors that yields a low P value. P-hacking is especially common now that many papers are pursuing small effects in noisy data sets.
The end result is that discoveries from exploratory research are treated as confirmations of effects. However, they are unlikely to replicate, especially if P-hacking is involved.
Any hopes for reformation will require a change in scientific culture and education. Statisticians should be encouraged not to report their own results as “significant” or “not significant”, but instead report effect sizes and confidence intervals. These answer the relevant questions of the magnitude and relative importance of a finding. Some are encouraging the scientific community to embrace Bayes rule. This requires a shift in thinking away from probability as a measure of the estimated frequency of an outcome to probability as a measure of the plausibility of an outcome. The advantage of the Bayesian approach is that scientists can incorporate what they already know about the world into their probability calculations (called “priors”). And they can calculate how probabilities change with the addition of new evidence (called “posteriors”).
Other suggested reforms include requiring scientists to explain omitted data and manipulations. This could reduce P-hacking if researchers are honest. Another idea is called “two-stage analysis.” It involves breaking a research project down into an exploratory phase, where interesting findings can be discovered and followed by a pre-registered confirmation phase. The replication would be published alongside the results from the exploratory study. Two-stage analysis could potentially reduce false positives, while still offering researchers flexibility.
The paper concludes by encouraging scientists to “realize the limits of conventional statistics.” They should be more willing to discuss the plausibility of their hypotheses and the limitations of their studies in research papers. A scientist should always ask three key questions after a study:
What is the evidence?
What should I believe?
What should I do?
One method of analysis cannot provide all of the answers.
P values are commonly used by scientists to see if something they're investigating is actually important or not. But, they might not be as trustworthy as many scientists think.
For example, a scientist named Matt Motyl in 2010 thought he had found out that people with extreme political views literally see the world in black and white. The P value was saying that this finding was very significant.
But when he and his mentor tried to do the study again, the P value wasn't even close to being significant. The idea he had found something important vanished.
The problem wasn't with the data or how it was analyzed. It was the P value itself. It's not really as reliable as most scientists might believe. Even some statisticians - people who study and work with things like P values - think they aren't always useful.
This can be a big problem when scientists are trying to see if their findings can be repeated, which is an important part of science. In fact, it's been suggested that most published scientific findings might actually be wrong. So, some statisticians are trying to find better ways to look at data that can help scientists avoid making mistakes or overlooking important stuff.
Despite all these issues, P values have been used for almost 90 years, so they're quite difficult to get rid of. In the 1920s, a man from the UK named Ronald Fisher introduced a concept called the 'P value'. He didn't mean for it to be a be-all-end-all type test, but rather a simple way to see if evidence was worth taking a closer look at. He wanted people to use it during experiments to check if their results were just random or if they really meant something.
The plan was to first come up with a guess, called a “null hypothesis”, that they wanted to prove wrong, like saying that there was no link or difference between two things or groups. Then, they would pretend that their null hypothesis was actually correct, and try to figure out what the odds were of getting the results they got, or something even more extreme. This chance they calculated was the P value. The smaller this value was, the more likely their null hypothesis was wrong.
Even though the P value seemed exact, Fisher only wanted it to be a part of a way to make scientific conclusions that mixed up data and general knowledge. Fisher had a few rivals who said his method was “worse than useless.” This movement led by Jerzy Neyman and Egon Pearson, recommended another way to analyze data, but left out the P value.
Because of their disagreements, other people got frustrated and started writing guides for scientists to use when dealing with statistics. Many of those authors didn't really understand either approach, so they just mixed them together and this is when a P value of 0.05 became the standard measure for 'statistically significant'. The P value wasn't intended to be used as it is today.
When researchers do an experiment and get a small P value, they often think it means they will get the same result if they do the experiment again.
But there's a problem. A P value of 0.01 doesn't mean there's a 1% chance of it being wrong. It also matters how likely the effect being studied was in the first place. If it is unlikely, then there is actually an 11% chance of it being wrong. So, if a scientist thought his experiment would work 99 out of 100 times, the truth could be closer to 73 out of 100 times.
Critics also say that P values can make researchers forget about the size of the effect they are studying. For instance, a study said people who met their spouses online were less likely to divorce and were happier in their marriages. But when looking closely, the actual differences between the groups were tiny..
Even worse, P values can be misused by researchers who keep testing until they get the results they want. This is called P-hacking. It can make things look like new discoveries even if they're just exploring.
So, the P value alone can't really tell us if an experiment's results are real, or important, or whether we're just playing with the numbers. P-hackingis often done in studies that look for tiny effects within a lot of messy information. It's hard to know how big this problem is, but many scientists think it's a major issue.
In one review, they found a suspicious behavior in psychology studies where too many P values gather near 0.05. It seems like researchers are cheating to get a significant P value.
However, changing the way we do statistics has been slow. This current way hasn't really changed since it was introduced by Fisher, Neyman, and Pearson. Some people have tried to discourage the use of P values in their studies, but this has been unsuccessful.
To reform, we need to change a lot of long-standing habits like how we're taught about statistics, how we analyze data and how we report and interpret results. At least now, many researchers are admitting that there is a problem.
Researchers think that instead of calling results "significant" or "not significant", they should talk about the size and importance of the effect. This tells us more than a P value does.
Some statisticians think we should replace the P value with a method from the 1700s, called Bayes rule, which thinks about probability based on how likely an outcome is. This method lets people use what they know about the world to understand their results and update their calculation of probability as new evidence is found.
The paper ends by asking readers to realize that their statistical methods have limits. One method alone can't answer all the important questions they are trying to find answers to.
--------- Original ---------
P values are often thought to be the 'gold standard' in scientific research to confirm whether an outcome is meaningful or significant. But they are not as trustworthy as many scientists think according to this paper by Regina Nuzzo.
For instance, in 2010, Matt Motyl, a psychology PhD student, thought he had made a big discovery. He believed he had found that people with extreme political views literally see the world in black and white, while those with moderate political views see more shades of gray. His data, based on a study of nearly 2,000 people, seemed to support this idea and his P value (0.01) indicated strong evidence for his claim.
But when he and his advisor, Brian Nosek, tried to reproduce the same results with new data, the P value increased to 0.59, implying that the result was not significant. This meant Motyl's findings could not be replicated.
The problem wasn't with Motyl's data or his way of analyzing it. Instead, it was the P value itself that was the issue. P values are not as reliable or unbiased as most scientists believe. Some statisticians have even suggested that most published findings based on P values could be inaccurate.
Another major concern revolves around whether or not other scientists can find similar results if they repeat the same experiment. If results can't be replicated, it challenges their validity. This has prompted many scientists to rethink how they evaluate their results, and statisticians to search for better ways of interpreting data.
P values have been used as a measure of validity in scientific research for almost 90 years, but they're actually not as dependable as many researchers think. Back in the 1920s, a UK statistician named Ronald Fisher invented the P value. He never intended for P values to be the final word on the significance of a finding, but rather a quick way to see if a result was worth further research. Here is the procedure for doing a P value analysis:
You start by making a “null hypothesis,” which predicts no effect or no relationship between two groups.
Then, playing the devil's advocate, you'd assume this null hypothesis is true and calculate the odds of getting results similar or more extreme than what you measured.
This chance is the P value. If it was small, Fisher suggested this probably meant your null hypothesis was wrong.
Fisher's rivals called his method “worse than useless.” Mathematician Jerzy Neyman and statistician Egon Pearson created their own way of analyzing data, leaving out the P value.
But while these rivals were arguing, others got tired of waiting and wrote manuals on statistics for working scientists. These authors didn't fully understand either approach, so they created a mixture of both. Fisher's easy-to-calculate P value was added into Neyman and Pearson's strict procedure. This is when a P value of 0.05 became accepted as 'statistically significant'.
This all led to a lot of confusion about what the P value really means. For example, if you got a P value of 0.01 in an experiment, most people think this means there's only a 1% chance your result is wrong. But that's incorrect. The P value can only summarize data based on a specific null hypothesis. It can't dig deeper and make statements about the actual reality. To do this, you need another piece of information: the odds that a real effect was there to begin with. Otherwise, you would be like someone who gets a headache and thinks it must mean they have a rare brain tumor, even when it's more likely to be something common like an allergy. The more unlikely the hypothesis, the greater the chance that an exciting finding is just a false alarm, regardless of the P value.
According to one “rule of thumb”, if your P value is 0.01, there's at least an 11% chance of a false alarm, and if your P value is only 0.05, the chance of a false alarm goes up to 29%. So, when Motyl did his research, there was more than a 1 in 10 chance his findings would not replicate. And there was only a 50% chance of getting as strong a result as in his original experiment.
Another problem with P values is how they can make us focus too much on whether there an effect exists, rather than on how big the effect is. Recently, there was a study of 19,000 people that found that people who met their spouses online are less likely to divorce and more likely to be happy in their marriage than those who met offline. This might sound like a big deal, but the effect was actually really small: meeting online decreased the divorce rate from 7.67% to 5.96%, and happiness only increased from 5.48 to 5.64 on a 7-point scale.
Even worse is something statisticians call "P-hacking", which means doing different calculations until you get the result you want. This skews the results toward what researchers hope to find. Some examples of P-hacking are leaving out some data to make a result stronger or checking the P value while data is being collected and then deciding whether to stop or gather more data.
The P value was never meant to be used like this. It makes a new discovery seem like solid evidence, but it is unlikely to be found again. Even small decisions made during analysis can raise the chances of a false positive to 60%. It has been noticed that a suspiciously high number of psychology studies have P values near 0.05, which suggests P-hacking was done to get some of these results.
Even though people have criticized P values for a long time, it has been hard to get rid of them. The editor of a psychology journal said back in 1982 that you can't convince authors to let go of their P values. The smaller they are, the harder the authors cling to them.
Experts have several ideas for how to improve the status quo.. For example, researchers should report the size and importance of an effect instead of just reporting their results as “significant” or “not significant.”
Some statisticians believe we should replace the P value with methods that use Bayes' rule. Bayes' rule is an 18th century idea that talks about probability as how likely something is to happen instead of how often it might happen. Using this method, researchers can incorporate what they know about a problem into their analysis and update their calculations as new data comes in.
The paper ends by encouraging scientists to “realize the limits of conventional statistics.” Scientists should always ask three key questions after a study:
What is the evidence?
What should I believe?
What should I do?
One method of analysis cannot answer all of these questions.
Homeschool:
Week 1:
1. Introduction to Statistics: Define the concept of 'P' values and why they are considered to be the 'gold standard' of statistical validity.
2. The Reliability of 'P' values: Discuss critically the assumption of many scientists that 'P' values are very reliable. Supplement this information with a case study, like the one in the text that discusses Matt Motyl and his study on political moderates and extremists.
Week 2:
3. Misconceptions of 'P' values: Use the story of Motyl and his follow-up study to illustrate that 'P' values might not always be indicative of accurate results, especially when trying to replicate a study.
4. Discussing Statistics Misuse: Share the story about statisticians issuing a warning over misuse of 'P' values. Converse about the potential issues this could cause within the scientific community.
Week 3:
5. In-depth Discussion on 'P' Values: Analyze the assertions by Stephen Ziliak about the limitations of 'P' values. Discuss why he feels they cannot properly do their job.
6. Additional Resources: Show a notable evidence or example about the reproducibility concerns, like the statement by epidemiologist John Ioannidis that most published findings are false.
Week 4:
7. Alternative Statistical Philosophies: Explore the perspectives of experts who are calling for a change in statistical philosophy, such as Steven Goodman. Analyze how a change in perspective changes what is important in statistical analysis.
8. Critics of 'P' values: Discuss criticisms that 'P' values have faced, including the metaphors used to describe them.
Week 5:
9. Recap and Review: Review all the lessons and key points learned about 'P' values, their reliability, and their criticisms.
10. Evaluation: Evaluate the lessons by testing the understanding of the child through questions and practical exercises. Give feedback based on their performance. Homeschool Curriculum: Understanding Statistical Significance
Week 1: Introduction to P Values and Hypothesis Testing
- Day 1: Learn about Ronald Fisher and his introduction of the P value in the 1920s
- Day 2: Discuss the purpose of P values and their role in experimental analysis - not as a definitive test, but as an informal measure of significance
- Day 3: Understand the concept of a 'null hypothesis' and explain its process
- Day 4: Review how to interpret P values
- Day 5: Weekly review and assessment
Week 2: Rivalry Over the Use of P Values
- Day 1: Study the works of Polish mathematician Jerzy Neyman and UK statistician Egon Pearson
- Day 2: Analyse differences between Fisher's attitude towards P values and that of Neyman and Pearson's alternative framework
- Day 3: Discuss how P values were integrated into Neyman and Pearson's rigorous, rule-based system
- Day 4: Understand the term 'statistically significant' and its relationship with P values
- Day 5: Weekly review and assessment
Week 3: Controversies in P Value Interpretation
- Day 1: Understand the confusion about P value meanings
- Day 2: Discuss common misconceptions about P values using the example of Motyl's study about political extremists
- Day 3: Learn why the P value alone can't make statements about underlying reality and why it requires additional information
- Day 4: Discuss how to correctly interpret findings from P values
- Day 5: Weekly review and assessment
Week 4: Conclusion and Assessment
- Day 1: Review the history and development of P values
- Day 2: Test knowledge on interpreting P values and their role in experimental analysis
- Day 3: Discuss issues related to the misuse of P values and possible solutions
- Day 4: Final review of all topics covered
- Day 5: Final assessment
Materials for the Course: Access to academic articles detailing works of Fisher, Neyman, and Pearson; examples of scientific studies using P values for analysis; exercises for calculating and interpreting P values.
Note: Encourage learners to question, analyse, and think critically about the information presented. This curriculum aims to develop not only knowledge about statistical significance but also critical thinking and analysis skills. Course Title: "Understanding Challenges in Irreproducible Research"
Course Description: This homeschool course aims to educate parents and children about the issues surrounding irreproducible research, focusing on the misinterpretation and misuse of P-values, and how biases can influence scientific outcomes.
Level: Advanced (High School)
Duration: 6 Weeks
Course Outline:
Week 1: Introduction to Statistics and Reproducibility in Research
Lesson 1: Understanding Basic Statistical Concepts
Lesson 2: The Importance of Reproducible Research
Activity: Discussion on the implications of irreproducible research
Week 2: Understanding P-Values
Lesson 1: What is a P-Value?
Lesson 2: Probability of False Alarms
Activity: Calculation exercises to understand P-Values
Week 3: Criticizing the Use of P-values
Lesson 1: How P-Values can Encourage Muddled Thinking
Lesson 2: Exploring Actual Size of an Effect Vs. P-Values
Activity: Case Study Analysis - "The Effect of Meeting Spouses Online on Divorce Rate"
Week 4: The Seductive Certainty of Significance
Lesson 1: Understanding the Seductive Certainty of Significance
Lesson 2: Determining Practical Relevance Vs. Statistical Significance
Activity: Discussion on the impact of misinterpreted statistics
Week 5: The Threat of P-Hacking
Lesson 1: Introduction to P-Hacking
Lesson 2: Understanding the concept of Data-Dredging, Significance-Chasing and Double-Dipping
Activity: Analysis of examples of P-Hacking
Week 6: The Misuse and Misunderstanding of P-Values in Research
Lesson 1: Exploring the Historical Context of P-value Usage
Lesson 2: Evaluating best practices in data analysis
Activity: Review and discussion on notable research studies where P-values were misused
Materials Needed:
- Statistics Calculator
- Access to online databases and references for case study analysis.
Recommended Prerequisite:
- Basic understanding of probability and statistics. Title: A Statistical Awareness Curriculum for Homeschooling Parents
Objective: Equip parents with the knowledge and tools to facilitate their children's understanding of statistics in today's research-saturated world.
Topics:
1. Introduction to the Importance of Statistics
- Understanding reasons for errors in data and 'hacking'
- The role of statistics in psychology research
2. The P-Value Paradox
- Understanding P values and their prevalence in research
- Discussing how P values influence research and why they can be problematic
3. History of Statistics in Research
- Looking at the influence of Fisher, Neyman, and Pearson
- Unpacking John Campbell's thoughts on P values
4. Changing Perspectives in Statistics
- An examination of how statistical practices have evolved over time
- Discussing the resurgence of P values despite criticism
5. Understanding Statistics in the Modern World
- How to understand and interpret P values in real-life research examples
- Analysis of the critiques of the current statistical framework
6. Calls for Statistical Reform
- Exploring needed changes in statistical teaching, analysis, and interpretation
- Discussing the implications of false findings
7. Proposed Solutions for Statistical Problems
- Discussing the importance of reporting effect sizes and confidence intervals
- Bayesian rule: understanding and applying
8. Incorporating Multiple Methods
- The importance of employing multiple analysis methods on the same dataset
- Practical examples to demonstrate the importance of diverse methods in statistics
Methods: Each topic would be delivered over a week, allowing learners to fully grasp the concepts. Parents would introduce the concepts using simple worksheets and interactive activities. The teaching aids would include real-life examples wherever possible to provide context. Children would be encouraged to apply the lessons learnt to everyday situations.
Assessment: Parents would assess their child's understanding through end-of-week quizzes and tests. They would also have discussions with their children to gauge their understanding. Text-based Curriculum: Science!
Week 1-2: Introduction to Science
Session 1: Understanding the Importance of Science
Exercise: Discuss the statement "Get the most important science stories of the day, free in your inbox." How is this relevant to our lives?
Session 2: Exploring Different Branches of Science
Activity: Explore the website 'Nature Briefing' (https://www.nature.com/briefing/signup/?brieferEntryPoint=MainBriefingBanner), read different articles daily for 1 week, and note down interesting discoveries.
Session 3: Sharing Discoveries
Activity: Choose a science story that fascinated you and share with the family why it impacted you.
Week 3-4: Visual Science: Understanding SVG (Scalable Vector Graphics)
Session 1: What is SVG?
Activity: Online research and presentation on "What is SVG and what are its applications in science?"
Session 2: Interpretation of SVG Data
Reading Assignment: Interpreting SVG data presented for an example image.
Note: Use the given SVG path data in the task for interpretation.
Session 3: Science in Visual Graphics
Practice Activity: Create a basic SVG graphic that represents a scientific concept.
Week 5-6: From Reading to Writing - Creating your Own Science Content
Session 1: Understanding How Science Stories are Written
Reading Activity: Paying attention to writing styles in 'Nature Briefing' articles. Note the structure, tone, and content.
Session 2: Creating Own Science Story
Writing Exercise: Based on your readings, create your own mini science story/article. Utilize SVG if possible.
Session 3: Peer Review
Activity: Exchange articles with your coursemates/family, read their work and provide constructive feedback.
Please remember, this is your home-schooling journey. Feel free to adapt and adjust as needed. Always focus on learning and exploration. Have fun!