Summary
In 2016, meta-researcher John Ioannidis and his colleagues published a paperanalyzing 385,000 studies and the abstracts of more than 1.6 million papers. Their findings show that use of the p-value has been increasing within research.
Ninety-six percent of the studies analyzed reported a significant p-value (less than 0.05) in the abstract. However, only ten percent of the reviewed studies reported effect sizes (a measure of the strength of a relationship between two variables) and confidence intervals (a measure of uncertainty). Without this context, p-values can be misleading. This is concerning because drugs and medical devices are justified based on the statistical significance of their p-values.
It is surprisingly hard to find a clear definition of a p-value. The American Statistical Association recently offered the following:
"Informally, a p-value is the probability under a specified statistical model that a statistical summary of the data (for example, the sample mean difference between two compared groups) would be equal to or more extreme than its observed value."
Researchers use p-values to help determine if differences between test groups are significant or not.
First, they define a null hypothesis, which predicts there will be no difference between the groups.
Next, the researcher calculates a p-value using their dataset.
Finally, a p-value is calculated, which tells the likelihood of the observed resultsassuming the null hypothesis is true.
A value less than 0.05 is generally interpreted to mean that the null hypothesis can be rejected. However, this does not tell the researcher anything about whether the drug worked or not.
The problem with p-values is they are often used to decide whether or not a study should be published in a journal. Good research could be rejected due to a high p-value. This may encourage researchers to game the system or selectively report results with a small p-value. This unethical practice is called p-hacking.
Ron Wasserstein of the American Statistical Association theorizes that the use of p-values has become widespread because they simplify a complex decision-making process into a single number. Wasserstein also notes that p-values are easy to calculate in the modern age due to software.
he p-value is being used for a purpose it was never intended for, according to Regina Nuzzo's reporting. UK statistician Ronald Fisher invented p-values in the 1920s to expedite the process of determining if a result warranted further examination. It was meant to be part of a larger process that “blended data and background knowledge to lead to scientific conclusions.” P-values were never meant to be the final word on significance.
In 2016, the American Statistical Association updated their guidelines on p-values:
P-values can indicate how incompatible the data are with a specified statistical model.
P-values do not measure the probability that the studied hypothesis is true, or the probability that the data were produced by random chance alone.
Scientific conclusions and business or policy decisions should not be based only on whether a p-value passes a specific threshold.
Proper inference requires full reporting and transparency.
A p-value, or statistical significance, does not measure the size of an effect or the importance of a result.
By itself, a p-value does not provide a good measure of evidence regarding a model or hypothesis.
In spite of his criticism, Ioannidis does not believe p-values should be banished from science. Instead, journals should insist on more information about “effect size, the uncertainty around effect size, and how likely [the results are] to be true.”
Many scientists use a measurement tool to decide if their expermint's results are noteworthy. This tool is called the p-value and it was invented in the 1920s. But some researchers are calling out an overuse of the p-value. They think it actually might be hurting science.
A 2016 study shows p-values are being used more often in research.. This could lead to potentially confusing, misleading, or even false findings in scientific experiments., Medicines or medical technology could pass inspection based on their “good” p-values – even if they don't actually work very well.
Researchers often use p-values to see if there is a difference between two groups. For example, one group who took a drug to lower cholesterol and another that didn't. The researcher wants to know if any difference in the groups was caused by the drug or some unknown factor. They use a p-value to decide if there is a real difference or not.
To calculate a p-value, a bunch of data about the study (like the number of people involved and the average change in their cholesterol levels) are plugged into a calculator. The
p-value cannot directly tell researchers if the medicine is working or not, but it can help them understand if the data from the study is unexpected. If the p-value is very low (less than 0.05), it means that getting such results by accident would be unlikely. But it cannot say anything about why there was a difference between the groups.
Scientists have several concerns about p-values. Some scientists use tricks to make their p-values look better so their studies can get published. There is also a concern that good studies aren't getting published because their p-values aren't low enough.
P-values are really common nowadays because they are so easy to calculate with a computer and they make it simple to decide what research is important. But this is a mistake. Even Ronald Fisher, the inventor of p-values, warned they should not be used this way. Instead, researchers should combine their data with what they already know about the world.
The American Statistical Association recently shared advice on how p-values should be used:
P-values only tell us how likely data is if the experiment doesn't have any effect.
P-values do not measure if an explanation of an effect is true or not.
Decisions in science, business, and politics should not be based on p-values.
Good research requires reporting all results honestly.
P-values do not measure the size of an effect or the importance of a result.
A p-value by itself can't say if a scientist's experiment has worked or not.
Even p-values biggest critics don't want to get rid of the tool altogether. . Instead, scientists need to talk more about the size of effects, what is unknown about an experiment, and how likely the research is to be true.
--------- Original ---------
Over the past couple of years, Stanford meta-researcher John Ioannidis and several colleagues have been working on a paper that should make any nerd think twice about p-values, those tests of statistical significance that are now commonly perceived as a signal of a study's worth. Their paper, published today in JAMA, examines p-values across 25 years of biomedical research. That involved doing some seriously impressive data crunching: The researchers analyzed more than 1.6 million study abstracts and more than 385,000 full-text papers, all of which included p-values.Researchers use a measurement tool called a p-valueto show that their research is significant.
The problem with p-values is that many people think a significant p-value proves a hypothesis, but that's not true. There's more factors in the mix, but scientists are increasingly relying on p-values to show their research is valid.
Scientist who overrely on p-values could end up with misleading study results. There's a fear that drugs and medical treatments could be promoted and sold if their p-values are “good” –even if the products don't work well.
The article explains that p-values are used when a researcher is trying to figure out if there is a difference between two groups being studied. For example, to find out if a drug lowers cholesterol, they have to find out if a group of people who took the drug is different from another group who didn't take the drug. Statistics cannot tell a researcher whether any difference between the groups was caused by the drug or other unknown factors. P-values help determine if the statistical difference between groups was likely or not.
Here's how it works:
The researcher defines a "null hypothesis", which predicts that the medicine doesn't affect cholesterol at all.
Then the p-value is calculated from the data you collected. It includes information like how many people were tested and the average changes in cholesterol.
The resulting p-value is a number between 0 and 1. A low p-value it is unlikely that the observed difference could have happened randomly. If the p-value is less than 0.05, many scientists consider the result "statistically significant", indicating the medicine is likely making a difference.
However, the p-value can't tell you if the medicine works or not. It just lets you know the odds of seeing this data if the medicine had no effect.
People are concerned that journals only publish studies with a p-value below 0.05. This means they sometimes ignore important research that doesn't have a low enough p-value. Even worse is that researchers might mess with their data to get low p-values, just to get published. This is called “p-hacking.”
P-values are everywhere because they are easy to calculate with a computer andmake it simple to decide whether a research finding is significant or not. But Ronald Fisher, the inventor of p-values, warned they should not be used this way. Instead, researchers should combine their data with what they already know about the world and make decisions based on a more fluid process.
The American Statistical Association recently shared advice on how p-values should be used:
P-values only tell us how likely data is if the experiment doesn't have any effect (the null hypothesis is true).
P-values do not measure if a hypothesis is true or not.
Decisions in science, business, and politics should not be based on p-values.
Good research requires reporting all results honestly.
P-values do not measure the size of an effect or the importance of a result.
A p-value by itself is not good evidence in favor of a hypothesis or explanation.
In spite of the criticism, this article does not say p-values should be eliminated. Instead, scientists need to talk more about the size of effects, what is unknown about an experiment, and how likely the research is to be true.
Homeschool:
Title: Understanding the Role of P-Values in Scientific Studies
Course Description:
This homeschooling curriculum is designed to help parents educate their children on the understanding and interpretation of p-values, their importance in scientific research, and the problems presented by the overreliance on p-values in science.
Course Outline:
1. Definitions and Fundamentals:
- What is a p-value?
- An Introduction to Hypothesis Testing
- Understanding Statistical Significance
2. The Role of P-values in Scientific Studies:
- Case Studies on P-value Usage in Biomedical Research
- Understanding Statistical Significance in Scientific Studies
- Interpreting P-values in Published Studies
3. Limitations and Misuse of P-values:
- Problems with Overreliance on P-values
- How P-values Can Be Misleading
- P-value: A Measure of Statistical Significance, not of Scientific Importance
4. Advancing Beyond P-values:
- Introduction to Effect Sizes and Confidence Intervals
- Importance of Giving Context to P-value Findings
5. P-values in Practical Application:
- Work through examples of hypothesis testing in biomedicine
- Analyse and interpret p-values from biomedical studies
Course Teaching Methods:
- Lecture-style teaching
- Online videos
- Interactive quizzes
- Problem-solving sessions
- Group discussions
- Hands-on practical tasks
At the end of this course, learners will understand the role of p-values in scientific research, recognize their limitations, and acquire the ability to critically assess their usage in scientific literature. Parents are provided with ample resources that make teaching this concept at home effective and engaging. Title: Understanding Hypothesis Testing and P-Value: A Homeschooling Guide for Parents
Objective: Equip parents with sufficient knowledge and tools to teach their children on hypothesis testing and p-value in a simplified manner.
Course Outcomes: By the end of the course, the learner should be able to;
- Understand the concept of the null hypothesis in hypothesis testing.
- Familiarize themselves with the process of generating a p-value.
- Calculate p-value independently using given data.
- Understand the interpretation of p-values in relation to the null hypothesis.
Course Breakdown:
Lesson 1: Introduction to Hypothesis Testing
- Define the concept of hypothesis testing.
- Explain the importance of hypothesis testing in scientific research.
Lesson 2: Understanding the Null Hypothesis
- Define the null hypothesis.
- Discuss examples of the null hypothesis in different scenarios.
Lesson 3: Introducing the P-value
- Define p-value and its role in supporting or rejecting the null hypothesis.
- Discuss examples of p-value calculations in different situations.
Lesson 4: How to Calculate P-values
- Discuss the necessary data needed to calculate a p-value.
- Teach the method of plugging in numbers into a calculator to obtain a p-value.
- Practice activity: Calculate p-values using given data.
Lesson 5: Interpreting the P-value
- Discuss what low and high p-values indicate regard to the null hypothesis.
- Discuss why a p-value of less than 0.05 is considered statistically significant in the medical community.
- Discuss the limitations of p-values in determining the truth or falsehood of the original hypothesis.
Lesson 6: P-values in Research Publication
- Discuss the role of p-values in determining research publishability.
- Discuss the potential negative impacts of overreliance on p-values on research validity such as "p-value hacking".
Conclusion: Responsible Use of P-values
- Discuss the guidance released by the American Statistical Association concerning more accurate and conservative use of the p-value.
Materials Needed: Internet access, calculators, sample data for exercise.
Additional Reading:
1. "An overview of Null hypothesis and P-value in Hypothesis Testing" by Ronald Fisher.
2. "Scientific Method and Statistical Errors" by Regina Nuzzo. Published by Nature. Homeschooling Curriculum: Practical Statistics for Parent Teachers
Week 1:
- Definition of Cholesterol and its importance in the body
- Cholesterol Levels and its impact on human health
Exercises:
- Research about different foods that can increase and decrease cholesterol.
Week 2 & 3:
- Introduction to Experiments: Group A (with medicine) vs Group B (without medicine)
- Understanding what variables and other factors are in experiments: Medicine and Cholesterol
Exercises:
- Find out real-life examples of experiments with two groups that differ in one or multiple variables.
Week 4 & 5:
- Understanding p-value: Introduction, Importance and its calculation
- Introducing Null Hypothesis: the Assumption for hypothesis and its role in p-value calculation
- Understanding "Statistically Significant" term and its relevance in the medical community
Exercise:
- Practice calculations of p-value with given numbers and data, understanding what is statistically significant
Week 6-8:
- Understanding limitations of the p-value: what it can and can't tell us
- Analysing the p-value concept critically: discussing Ioannidis's paper about the crisis of p-value
Exercises:
- Read and understand Ioannidiss paper. Respond with individual thoughts and analysis.
Week 9-10:
- Concept of "P-dolatory or the "worship of false significance"
- Understanding how p-values are used or misused in research publications
- Discussing about "p-value hacking"
Discussion:
- Discuss about the declare of p-value misuse by Ron Wasserstein, the executive director of the American Statistical Association.
Week 11 & 12:
- Reflecting on the role of technology in p-value calculations
- Understand the reliance on p-value in determining the value of research
Exercise:
- Discuss real-life cases where the p-value was heavily relied on in research. Debate over whether this dependency is justified.
Throughout this course, we will make use of real-life examples, engage in critical thinking discussions and provide practical exercises to understand statistical methods and their importance in the world of science and medicine.
--------- Original ---------
Over the past couple of years, Stanford meta-researcher John Ioannidis and several colleagues have been working on a paper that should make any nerd think twice about p-values, those tests of statistical significance that are now commonly perceived as a signal of a study's worth. Their paper, published today in JAMA, examines p-values across 25 years of biomedical research. That involved doing some seriously impressive data crunching: The researchers analyzed more than 1.6 million study abstracts and more than 385,000 full-text papers, all of which included p-values.Let's start with the truth!
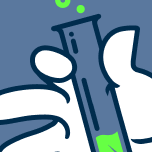
Support the Broken Science Initiative.
Subscribe today →
recent posts
Metabolic Flexibility to Burn Fat, Get Stronger, and Get Healthier
Expanding Horizons: Physical and Mental Rehabilitation for Juveniles in Ohio