By William Briggs
Summary
' En este artículo, el estadístico William Briggs analiza un estudio que buscaba cuantificar el impacto global de las vacunas COVID-19 en el primer año en que estuvieron disponibles (2021). Briggs argumenta que el modelo matemático del estudio es defectuoso porque asume que las vacunas COVID funcionan, en lugar de probar si lo hacen. El modelo asume que las vacunas reducen las infecciones graves que conducen a la hospitalización o la muerte y previenen la transmisión. Sin embargo, el modelo no tiene en cuenta las infecciones de COVID previas, que eran generalizadas cuando se lanzaron las vacunas. Esto significa que el modelo probablemente sobrestima la eficacia de la vacuna al atribuir la reducción de muertes a las vacunas, pero ignora el impacto de la inmunidad natural adquirida mediante una infección anterior. Briggs también critica el uso del estudio de "muertes excesivas" por parte del estudio, que compara el número real de muertes en un año determinado con el número de muertes predicho por un modelo. Además, el modelo asume que todas las muertes excesivas estuvieron relacionadas con COVID. Muchos de los factores que llevaron a un mayor número de muertes excesivas en 2020, como el pánico, los efectos adversos de los cierres y el tratamiento excesivamente agresivo en los primeros casos de COVID, se redujeron en 2021, el año de este estudio. Al no tener en cuenta la reducción de estos factores, el estudio probablemente sobrestima la eficacia de la vacuna. Muchas fuentes de incertidumbre fueron ignoradas por este estudio. Las muertes excesivas son una estimación con un nivel de error. Pero esta incertidumbre no se tuvo en cuenta en su cálculo del número de vidas salvadas por la vacuna. El análisis país por país de este documento también ignora muchas diferencias importantes entre los países, como la edad y la salud de sus poblaciones, las políticas gubernamentales, diferentes vacunas y más. En general, Briggs sostiene que al omitir muchos puntos de datos importantes, el estudio probablemente exagera la eficacia de la vacuna. Debido a los muchos factores confusos presentados aquí, es casi imposible cuantificar con precisión la eficacia de la vacuna.'
'Este artículo de William Briggs examina un estudio que intenta explicar cómo las vacunas contra el COVID-19 salvaron vidas alrededor del mundo en su primer año de uso. Los científicos que escribieron el estudio hicieron un modelo matemático para estimar cuán bien funcionaron las vacunas. Pero Briggs señala que su modelo no pudo decir precisamente cuán bien funcionaron las vacunas. En primer lugar, el modelo simplemente asumió que las vacunas funcionaban. No probó realmente si lo hacían. El modelo tampoco observó cuántas personas ya tenían COVID antes de recibir la vacuna. Muchas personas tuvieron COVID antes de ser vacunadas. Muchas no sabían que lo tenían porque estaban sanas y nunca desarrollaron síntomas. De cualquier manera, desarrollaron inmunidad natural que puede ayudar a prevenir una infección en el futuro o hacerla menos grave. Debido a este error, el modelo pudo haber hecho parecer que la vacuna salvó más vidas de las que realmente hizo. El modelo utilizó "muertes excesivas" para medir cuán bien funcionaba la vacuna. Las muertes excesivas comparan el número real de muertes en un año con una predicción de cuántas muertes ocurrirían en un año “normal” para esa población. El modelo asumió que todas estas muertes adicionales eran por COVID. Las muertes excesivas podrían ser por otras causas como el pánico, los cierres y los tratamientos perjudiciales para el COVID, como se vio el año anterior. En 2021, las muertes excesivas disminuyeron, y el modelo atribuyó ese descenso a las vacunas funcionando. Pero el modelo no tuvo en cuenta todas las otras razones, llamadas variables de confusión, que podrían haber llevado a esta disminución en las muertes excesivas. El modelo también ignoró variables de confusión como las diferencias entre países, incluyendo qué vacunas usaron, sus políticas gubernamentales, y la edad y la salud de sus poblaciones. Esto agrega aún más incertidumbre a si las vacunas previnieron las muertes excesivas o no. En resumen, el modelo utilizado en el estudio tenía serios defectos. Probablemente hizo que las vacunas parecieran más efectivas de lo que eran. El modelo era demasiado simple para capturar esto. Al ignorar muchas variables de confusión, este estudio nos deja con más preguntas que respuestas y no debería usarse como evidencia de que estas vacunas contra el COVID-19 funcionan.'
--------- Original ---------
'El estadístico William Briggs escribió este artículo sobre un estudio que afirmaba medir el efecto de las vacunas COVID-19. Advierte que varios factores omitidos en el análisis hacen que la vacuna parezca funcionar mejor de lo que realmente lo hizo. Sin los factores adicionales, no deberíamos estar convencidos de que estas vacunas funcionan como afirma este estudio. Briggs señala que hay muchas variables de confusión que deben abordarse para hacer cualquier afirmación sobre cuán bien funcionan las vacunas. Las variables de confusión son factores que pueden afectar el resultado de un estudio, pero que los investigadores no tuvieron en cuenta. Una variable de confusión muy importante es el efecto de las infecciones previas. Para cuando se distribuyeron las vacunas en 2021, muchas personas ya habían adquirido inmunidad natural a partir de una infección previa. Muchas personas que recibieron la vacuna ni siquiera sabían que habían sido infectadas anteriormente. En cualquier caso, su inmunidad natural podría ayudar a prevenir o reducir la gravedad de futuras infecciones por COVID-19, además del efecto que pueda tener la vacuna. Briggs desafía la suposición de que las muertes excesivas medidas en el estudio fueron causadas por COVID-19. Las muertes excesivas es una comparación de cuántas personas murieron en un año determinado y cuántas se esperaría que murieran en un año "normal", según lo predice otro modelo. Briggs afirma que el documento no consideró otros factores, como el pánico, los bloqueos y los tratamientos COVID demasiado agresivos que podrían haber aumentado las muertes excesivas en el año anterior. Atribuir todas las mejoras en las muertes excesivas a la vacuna, dice, podría llevar a afirmaciones exageradas sobre la eficacia de la vacuna. Si bien las vacunas pueden ayudar a las personas, también hay riesgos propios de la vacuna. Estos pros y contras deben ser sopesados por los pacientes y los cuidadores. Briggs también señala que hay muchas incertidumbres al mirar esto a escala global porque hay diferencias en cómo respondió cada país, los tipos de vacunas utilizadas y la edad y la salud de la población. En conclusión, Briggs señala lo difícil que es juzgar con precisión cuán efectiva fue la vacuna COVID-19 en 2021. Simplemente hay demasiados factores importantes que este estudio no logra tener en cuenta, incluyendo infecciones previas, diferencias entre países e incertidumbre en las mediciones de muertes excesivas, que no es posible para los autores hacer una estimación precisa de la efectividad de la vacuna COVID-19.'
--------- Original ---------
How can you tell if a vaccine for a bug is effective? It’s not so easy; indeed, it can be excruciatingly difficult.
At the individual person level you’d need to measure all kinds of things, like the level of antibodies and other immune cells present before vaccination, and then again after and through time.
Then you’d demonstrate, in that person, the exact mechanism by which the vaccine was able to boost immunity, and whether this boost was sufficient to quell the infection, by looking at severity of illness (due to the bug and other existing conditions), how long it took for the infection to abate, and things like that. And that is only a hint of the complexities.
The analysis is made harder because the vaccinated person may never come into contact with the virus. People he meets may have already had prior infections, and so are now mostly or completely immune. Or those people have had a vaccine that was effective to varying degrees.
As difficult as all that sounds, it is not impossible in highly controlled circumstances to discover the extent and to quantify vaccine effectiveness. But it is a slow and painstaking process.
One way you cannot learn, not with anything approaching certainty, is looking at group-level comparisons, where people are not individually counted and compared, but where averages across groups are contrasted, and where you have no idea what the status of any individual is.
This is a popular kind of analysis because it’s cheap and easy. But it can, and often does, lead to huge over-certainties.
A prime example is from the paper “Global impact of the first year of COVID-19 vaccination: a mathematical modelling study” by Oliver J Watson, Gregory Barnsley, Jaspreet Toor, Alexandra B Hogan, Peter Winskill, and Azra C Ghani, in Lancet Infectious Disease.
They used a “mathematical model of COVID-19 transmission and vaccination” for both “reported COVID-19 mortality and all-cause excess mortality in 185 countries and territories” to assess vaccine efficacy in preventing deaths. This is as group-level an analysis as they come, especially with its “excess” deaths portion.
What are “excess” deaths? Deaths different than those predicted by a model. They can be positive, meaning more deaths than predicted by a model, or negative, meaning fewer deaths than predicted by a model. What it means here is that Watson and his co-authors used as input to their model, output from another model, and what that means I’ll explain in a moment.
Let’s first look at their transmission model.
In it, “Vaccination was assumed to confer protection against SARS-CoV-2 infection and the development of severe disease requiring hospital admission, and to reduce transmission from vaccine breakthrough infections”. Incidentally, “Breakthrough infection” is a term of incredulity: it assumes vaccines work and that, somehow, bugs are able to bypass it sometimes.
In other words, their model was told that covid vaccination worked. The model was told that the vax blocked infection and prevented severe disease, including death, and the model was told that infections were harder to pass on in the vaccinated.
The only thing this model can “discover”, therefore, is that the covid vaccine works. It could do nothing else.
Now about the data they used: “The first vaccination outside a clinical trial setting was given on Dec 8, 2020. We introduced vaccination from this point onwards in the model and explored the impact of the first year of vaccination up to Dec 8, 2021.”
Vaccinations did not begin in earnest, however, until spring of 2021, with a peak number of shots in April 2021. The bug, and its early mutations, had been circulating for at least a year by that point. This means many people were already infected by the spring of 2021. And many, the CDC estimated about 15%, never even knew they were infected.
Therefore the biggest flaw in this, and many other analyses, is not accounting for prior infection. This is an immense problem because we can not tell by looking at group-level data whether changes in infections and illness (including death) were caused by prior unnoticed infections or because of vaccinations.
Because of the panic, the majority of people who sought vaccination never had their background covid antibodies checked. After all, you could get the vaccine at the local drug store. It’s possible some of those with prior infections and who got the vax had superior immunity to those without either, but it’s also possible for some the addition of the vax on top of prior infection did nothing.
If this is confusing, what it means is that this, or any, analysis that does not account for previous infection will overestimate, perhaps by a lot, vaccine efficacy. Because it will credit the vax for deaths that were prevented by previous infection. There is also the possibility that other “unapproved” treatments, such as vitamin D and ivermectin, which many took in 2021 also would give credit to the vax.
The authors then fit their same model to “excess” deaths. There are two major flaws with this.
The first is assuming all “excess” deaths were due to or related to covid. This is almost certainly false, as I analyze here. There were still large numbers of deaths above what was usually expected (that “usually expected” is a model) after subtracting covid deaths, especially early in the panic.
These could be caused by aggressive over-treatment for covid, lockdowns, and people too scared to have other life-threatening conditions checked out, especially in 2020 when the panic was at its peak.
The initial frenzied panic subsided somewhat in 2021, which means many “excess” deaths decreased merely from lessened panic. This, too, is credited to the vax in their model.
The second mistake, even allowing that all “excess” deaths were indeed covid related, is that the uncertainty in the estimates of “excess” deaths—there is a plus-or-minus for these—was not incorporated into the estimated number of lives saved by the vax. This means that their own plus-or-minus windows for lives saved will be far too narrow.
None of these criticisms mean that the vaccine did not offer some protection in some people, while also conferring some risk of injury from the vaccination itself. Our point is that there are massive uncertainties that were not accounted for, leading to an exaggerated view of the vaccine’s efficacy.
A further uncertainty is the global nature of this analysis. Countries varied widely in behavior to and in the panic. Vaccines differed. Background health differed. Official policy differed. Really, most things of important differed. Numbers are all over the map. A gross country-by-country analysis is leaving far too much uncertainty unaccounted for.
Especially because of the blind panic, and the failure to note prior infection, it will forever be difficult, or even impossible, to know just how efficacious the vaccine was in 2021.
I am a wholly independent writer, statistician, scientist and consultant. Previously a Professor at the Cornell Medical School, a Statistician at DoubleClick in its infancy, a Meteorologist with the National Weather Service, and an Electronic Cryptologist with the US Air Force (the only title I ever cared for was Staff Sergeant Briggs).
My PhD is in Mathematical Statistics: I am now an Uncertainty Philosopher, Epistemologist, Probability Puzzler, and Unmasker of Over-Certainty. My MS is in Atmospheric Physics, and Bachelors is in Meteorology & Math.
Author of Uncertainty: The Soul of Modeling, Probability & Statistics, a book which calls for a complete and fundamental change in the philosophy and practice of probability & statistics; author of two other books and dozens of works in fields of statistics, medicine, philosophy, meteorology and climatology, solar physics, and energy use appearing in both professional and popular outlets. Full CV (pdf updated rarely).
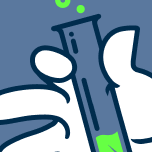
Support the Broken Science Initiative.
Subscribe today →
One Comment
Leave A Comment
You must be logged in to post a comment.
recent posts
Greg Joins Chris Cooper’s Two-Brain Business Podcast
Education from Dr Sarah Hallberg’s TEDx talk
This is my first time pay a quick visit at here and i am really happy to read everthing at one place