Summary
This editorial commentary by Gerd Gigerenzer and Julian Marewski discusses the dream of a universal method of inference in science. The great mathematician Gottfried Wilhelm Leibniz dreamed of a universal calculus in which all ideas could be represented by symbols and discussed without bickering. He predicted the project would take five years, but alas, has never been completed. In its stead, a surrogate, the p-value, has been adopted as the preferred method of inference for scientific research. Critics have argued that Bayesian methods are superior and should be the only means of analysis going forward.
The authors of this paper argue that both groups are wrong in seeking the “false idol” of a universal method of inference. P-values have done great damage by replacing actual replications with statistical inferences that purport to estimate replicability. Large efforts to conduct replications of landmark studies have shown that surprisingly few replicate in spite of small p-values. Amgen scientists were unable to replicate 47 of 53 experiments that provided targets for potential breakthrough drugs. These were considered to be major studies and Amgen had a financial interest in making them work. Another analysis of replications in management, finance, and advertising journals showed that 40-60% of replications contradicted the results of the original studies.
Statisticians have warned about p-values for as long as they have existed, but they are still prevalent. One researcher calculated the average number of p-values reported in papers published in a business journal. It was 99. The authors even found a p-value and confidence interval calculated for the number of subjects in a study, as if there could be any uncertainty about that! This is simply the mindless application of statistics made easy by user-friendly statistics software.
Bayesians on the other hand were initially careful to apply their methods only to “small world” situations where uncertainty is minimal. Later on, their confidence in the method grew and they proposed “universal Bayesianism” that can apply to statistical repetitive events or singular events. The authors of this paper suggest that Bayes theorem can be useful for determining risk, but is of uncertain value in an uncertain world.
The authors call the automatic use of Bayes rule in science a “beautiful idol,” but warn that it should only be one tool in a larger toolbox. An example of the successful application of Bayes in medicine is calculating the probability that someone has a disease when they have tested positive and the background prevalence of the disease is known. Without an objective prior, such as the reliably estimated prevalence of a disease, Bayes becomes more subjective and less reliable.
The authors have three key points to make in this commentary:
There is no universal method of scientific inference, but rather a whole toolbox which includes descriptive statistics, exploratory data analysis, and formal modeling techniques. The only thing that doesn't belong in the toolbox are false idols.
If the hoped for “Bayesian Revolution” takes place, one false idol could be swapped for another. Bayes factors might simply replace p-values as the automatically calculated significance level of science.
The methods of statistical analysis used in science change science itself, and vice-versa. For instance, as the social sciences increasingly emphasized inferential statistics, the importance of replication and measurement error diminished.
Past scientific breakthroughs, such as by Isaac Newton and Charles Darwin, made no use whatsoever of inferential statistics. Newton conducted careful experiments to demonstrate the effects predicted by his theories. No statistics were reported, even though he was familiar with statistical methods and even employed them for quality control in his occupation as master of the London Royal Mint. In the field of psychology, now dominated by inferential statistics, earlier breakthroughs by Piaget, Pavlov, Skinner and others made no use of them either. Neither statistical nor Bayesian inference played any major role in science until the 1940s.
Not soon after, the “Null Ritual” became the predominant method of inference, especially in the social sciences. It consists of three steps:
Set up a null hypothesis (no mean difference or zero correlation) without stating your own hypothesis.
Use 5% as a convention for rejecting the null.
Always perform this procedure. This ritual became codified in scientific publication manuals, which determined the style and substance of published research.
The null ritual is a hybrid of the work of Ronald Fisher and Neyman/Pearson, though it violates the recommendations of both. Fisher once proposed the 5% threshold as a convention, but later disavowed this practice. He advised that researchers should:
set up a null hypothesis that is not necessarily a nil hypothesis.
Report the exact level of significance found.
Use this procedure only if little is known about the problem being studied.
The authors conclude their editorial with the grim admission of one benefit of the null ritual: a steady source of employment for average scientists who will continue to publish uncreative research with little innovation or risk.
One great dream of scientists is to one day have a way to analyze any research and easily figure out if it is reliable knowledge or not. However, no one has ever accomplished this goal, but many scientists have acted as if they have a universal method.
Their favored method is called a p-value. It is believed that a small p-value means that it is more than 95% likely that the result they find in an experiment will happen again if they repeat the experiment. However, when scientists redo the experiments of other scientists, they are failing to get the same result in more than half the time. This means that a lot of scientific knowledge is not as accurate as we once thought.
A big part of the problem is that scientists have software that makes it easy to find a p-value for whatever data they plug in to their software. These scientists are not aware that other methods might be better for the type of research they are analyzing. Instead, they are following what the authors of this editorial call the null ritual.
The null ritual is when data is compared to an arbitrary null hypothesis (the expectation of no effect or correlation). If the data gets a small p-value, then the null hypothesis is rejected in favor of the researcher's hypothesis (the expectation of the researcher's idea being tested). And the last part of the ritual is to always perform this procedure.
Other researchers have promoted a method called Bayes' theorem, which they think is the only way to analyze research. There are some really effective applications of Bayes, like figuring out if someone is really sick when they have tested positive for a disease. If it is a rare illness, there is a good chance the patient is fine and the test was wrong (a false positive in this case).
The authors of this paper advise that scientists should become familiar with a wider variety of statistical methods and use each tool from this toolbox when it is the right one for the job at hand. Until then, scientists will continue to produce tons of average-quality research.
--------- Original ---------
"The application of statistics to science is not a neutral act. Statistical tools have shaped and were also shaped by its objects. In the social sciences, statistical methods fundamentally changed research practice, making statistical inference its centerpiece. At the same time, textbook writers in the social sciences have transformed rivaling statistical systems into an apparently monolithic method that could be used mechanically. The idol of a universal method for scientific inference has been worshipped since the “inference revolution” of the 1950s. Because no such method has ever been found, surrogates have been created, most notably the quest for significant p values. This form of surrogate science fosters delusions and borderline cheating and has done much harm, creating, for one, a flood of irreproducible results. Proponents of the “Bayesian revolution” should be wary of chasing yet another chimera: an apparently universal inference procedure. A better path would be to promote both an understanding of the various devices in the “statistical toolbox” and informed judgment to select among these."In the 1600s, a famous mathematician named Gottfried Wilhelm Leibniz proposed a search for a universal method of analyzing information. He thought it would only take five years, but this project has still never been completed. But many scientists act as if they have a universal method and they apply it to every research problem they can.
The most common method of statistical analysis in science, especially the social sciences like psychology, management and finance, is called a p-value. Most scientists think a small p-value means there is a high likelihood (over 95%) that their research findings will replicate if their experiment is repeated. However, many large-scale replication projects have shown that greater than half of published science fails to replicate. A major biotech company called Amgen failed to replicate 47 of 53 major academic studies that had proposed possible new drug treatments for cancer. A lot of time and money has been wasted pursuing research based on unreliable science.
The authors of this paper write that scientists blindly follow a “null ritual” when it comes to interpreting the results of their experiments. First, they compare their results to a null hypothesis. The null is an expectation of zero correlation or effect. Second, they set an arbitrary threshold of 5% to determine if their findings are significant. If this threshold is cleared, they reject the null hypothesis in favor of their own hypothesis. And third, they repeat this process in every experiment. This ritual has been taught in statistics textbooks for psychologists and social scientists and required by many publishers.
Even the inventor of hypothesis testing, Ronald Fisher, advises against this procedure. He says that a threshold for significance should be different for every research project. A null hypothesis doesn't always have to be a zero correlation. And this procedure should not be done all the time.
Many great scientists in the past, like Isaac Newton and Charles Darwin, never used p-values or any type of inferential statistics. Most of the breakthroughs in science up till the 1940, including the social sciences, didn't report p-values or confidence intervals or any of the statistics that are seen all over the place today. Instead, great scientists like Newton, Pavlov, and Skinner conducted experiments to demonstrate the effects predicted by their theories.
The authors of this paper advise against the search for a universal method of analysis. They call it a “false idol.” This includes a popular method called Bayes' theorem. It can be very useful in some situations where there is little uncertainty, but it should not be applied automatically in all situations. One example of where it is useful is in medical diagnosis. If a person has tested positive for a disease, it is not necessarily true that a person actually has the disease, even if the test is very reliable. If the disease is rare in the population, then there is a good chance that most people who test positive actually don't have the disease. In this case, false positives are more common than true positives.
The authors conclude that scientists need to learn a wide variety of statistical methods and know when each one is the appropriate tool for the job. Otherwise, scientists will continue to pump out research that is of average quality and frequently fails to replicate.
--------- Original ---------
"The application of statistics to science is not a neutral act. Statistical tools have shaped and were also shaped by its objects. In the social sciences, statistical methods fundamentally changed research practice, making statistical inference its centerpiece. At the same time, textbook writers in the social sciences have transformed rivaling statistical systems into an apparently monolithic method that could be used mechanically. The idol of a universal method for scientific inference has been worshipped since the “inference revolution” of the 1950s. Because no such method has ever been found, surrogates have been created, most notably the quest for significant p values. This form of surrogate science fosters delusions and borderline cheating and has done much harm, creating, for one, a flood of irreproducible results. Proponents of the “Bayesian revolution” should be wary of chasing yet another chimera: an apparently universal inference procedure. A better path would be to promote both an understanding of the various devices in the “statistical toolbox” and informed judgment to select among these."Let's start with the truth!
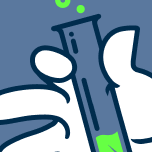
Support the Broken Science Initiative.
Subscribe today →
recent posts
Expanding Horizons: Physical and Mental Rehabilitation for Juveniles in Ohio
Maintaining quality of life and preventing pain as we age.