By William Briggs
Summary
In this article, statistician William Briggs analyzes a study that aimed to quantify the global impact of COVID-19 vaccinations in the first year they were available (2021). Briggs argues the study's mathematical model is flawed because it assumes COVID vaccines work, rather than testing if they do. The model assumes that vaccines reduce severe infections leading to hospitalization or death and prevent transmission. However, the model does not account for prior COVID infections, which were widespread by the time vaccines rolled out. This means the model likely overestimates vaccine efficacy by attributing reduced deaths to vaccines, but ignores the impact of natural immunity acquired via an earlier infection.
Briggs also critiques the study's use of “excess deaths,” which compares the actual number of deaths in a given year to the number of deaths predicted by a model. Further, the model assumes all excess deaths were COVID-related. Many of the factors that led to higher excess deaths in 2020–such as the panic, the adverse effects of lockdowns, and over-aggressive treatment in early COVID cases–were reduced in 2021, the year of this study. By not accounting for the reduction in these factors, the study likely overestimates the efficacy of the vaccine.
Many sources of uncertainty were ignored by this study. Excess deaths are an estimate with a level of error. But this uncertainty was not factored into their calculation of the number of lives saved by the vaccine. The country-by-country analysis of this paper also ignores many important differences between countries, such as the age and health of their populations, government policies, different vaccines, and more.
Overall, Briggs contends that by leaving out a lot of important data points, the study likely exaggerates vaccine efficacy. Due to the many confounding factors presented here, it is nearly impossible to accurately quantify vaccine efficacy.
This article by William Briggs examines a study that attempts to explain how the COVID-19 vaccines saved lives around the world in their first year of use.
The scientists who wrote the study made a math model to estimate how well the vaccines worked. But Briggs points out that their model could not say precisely how well the vaccines worked.
First, the model just assumed the vaccines worked. It didn't actually test if they did. The model also didn't look at how many people already had COVID before getting the vaccine. Lots of people had COVID before they were vaccinated. Many didn't know they had it because they were healthy and didn't ever develop symptoms. Either way, they developed natural immunity which can help prevent an infection in the future or make it less severe. Because of this error, the model might have made it seem like the vaccine saved more lives than it actually did.
The model used "excess deaths” to measure how well the vaccine worked. Excess deaths compares the actual number of deaths in a year to a prediction of how many deaths would occur in a “normal” year for that population. The model assumed all these extra deaths were from COVID. Excess deaths could be from other causes like the panic, lockdowns, and harmful treatments for COVID, as were seen the previous year.
In 2021, excess deaths went down, and the model attributed that decline to vaccines working. But the model didn't account for all the other reasons, called confounding variables, that might have led to this decrease in excess deaths. The model also ignored confounding variables like the differences between countries including which vaccines they used, their government policies, and the age and health of their populations. This adds even more uncertainty to whether or not the vaccines prevented excess deaths.
In summary, the model used in the study had serious flaws. It likely made the vaccines look more effective than they were.The model was too simple to capture this. By ignoring many confounding variables, this study leaves us with more questions than answers and it should not be used as evidence that these COVID-19 vaccines work.
--------- Original ---------
Statistician William Briggs wrote this piece on a study which claimed to measure the effect of COVID-19 vaccines. He warns that several factors left out of the analysis make the vaccine appear to work better than it really did. Without the additional factors we should not be convinced these vaccines work as this study claims.
Briggs points out that there are many confounding variables that need to be addressed in order to make any claims about how well vaccines work. Confounding variables are factors that may affect the outcome of a study, but were not accounted for by the researchers.
One very important confounding variable is the effect of previous infections. By the time vaccines rolled out in 2021, many people had already acquired natural immunity from a previous infection. Many people who received the vaccine didn't even know they had been previously infected. In any case, their natural immunity could help prevent or reduce the severity of future COVID-19 infections, in addition to whatever effect the vaccine has.
Briggs challenges the assumption excess deaths measured in the study were caused by COVID-19. Excess deaths is a comparison of how many people died in a given year and how many would be expected to die in a “normal” year, as predicted by another model. Briggs asserts that the paper didn't consider other factors, like the panic, lockdowns, and overly aggressive COVID treatments that could have increased excess deaths in the previous year. Attributing all improvements in excess deaths to the vaccine, he says, could lead to exaggerated claims of the vaccine's effectiveness. While vaccines can help people, there are also risks from the vaccine itself. These pros and cons must be weighed by patients and caregivers.
Briggs also points out that there are many uncertainties when looking at this on a global scale because there are differences in how each country responded, the types of vaccines used, and the age and health of the population. In conclusion, Briggs points out just how difficult it is to accurately judge how effective the COVID-19 vaccine was in 2021. There are simply too many important factors this study fails to account for–including previous infections, differences between countries, and uncertainty in excess death measurements–that it is not possible for the authors to make any precise estimation of the effectiveness of the COVID-19 vaccine.
--------- Original ---------
How can you tell if a vaccine for a bug is effective? It’s not so easy; indeed, it can be excruciatingly difficult.
At the individual person level you’d need to measure all kinds of things, like the level of antibodies and other immune cells present before vaccination, and then again after and through time.
Then you’d demonstrate, in that person, the exact mechanism by which the vaccine was able to boost immunity, and whether this boost was sufficient to quell the infection, by looking at severity of illness (due to the bug and other existing conditions), how long it took for the infection to abate, and things like that. And that is only a hint of the complexities.
The analysis is made harder because the vaccinated person may never come into contact with the virus. People he meets may have already had prior infections, and so are now mostly or completely immune. Or those people have had a vaccine that was effective to varying degrees.
As difficult as all that sounds, it is not impossible in highly controlled circumstances to discover the extent and to quantify vaccine effectiveness. But it is a slow and painstaking process.
One way you cannot learn, not with anything approaching certainty, is looking at group-level comparisons, where people are not individually counted and compared, but where averages across groups are contrasted, and where you have no idea what the status of any individual is.
This is a popular kind of analysis because it’s cheap and easy. But it can, and often does, lead to huge over-certainties.
A prime example is from the paper “Global impact of the first year of COVID-19 vaccination: a mathematical modelling study” by Oliver J Watson, Gregory Barnsley, Jaspreet Toor, Alexandra B Hogan, Peter Winskill, and Azra C Ghani, in Lancet Infectious Disease.
They used a “mathematical model of COVID-19 transmission and vaccination” for both “reported COVID-19 mortality and all-cause excess mortality in 185 countries and territories” to assess vaccine efficacy in preventing deaths. This is as group-level an analysis as they come, especially with its “excess” deaths portion.
What are “excess” deaths? Deaths different than those predicted by a model. They can be positive, meaning more deaths than predicted by a model, or negative, meaning fewer deaths than predicted by a model. What it means here is that Watson and his co-authors used as input to their model, output from another model, and what that means I’ll explain in a moment.
Let’s first look at their transmission model.
In it, “Vaccination was assumed to confer protection against SARS-CoV-2 infection and the development of severe disease requiring hospital admission, and to reduce transmission from vaccine breakthrough infections”. Incidentally, “Breakthrough infection” is a term of incredulity: it assumes vaccines work and that, somehow, bugs are able to bypass it sometimes.
In other words, their model was told that covid vaccination worked. The model was told that the vax blocked infection and prevented severe disease, including death, and the model was told that infections were harder to pass on in the vaccinated.
The only thing this model can “discover”, therefore, is that the covid vaccine works. It could do nothing else.
Now about the data they used: “The first vaccination outside a clinical trial setting was given on Dec 8, 2020. We introduced vaccination from this point onwards in the model and explored the impact of the first year of vaccination up to Dec 8, 2021.”
Vaccinations did not begin in earnest, however, until spring of 2021, with a peak number of shots in April 2021. The bug, and its early mutations, had been circulating for at least a year by that point. This means many people were already infected by the spring of 2021. And many, the CDC estimated about 15%, never even knew they were infected.
Therefore the biggest flaw in this, and many other analyses, is not accounting for prior infection. This is an immense problem because we can not tell by looking at group-level data whether changes in infections and illness (including death) were caused by prior unnoticed infections or because of vaccinations.
Because of the panic, the majority of people who sought vaccination never had their background covid antibodies checked. After all, you could get the vaccine at the local drug store. It’s possible some of those with prior infections and who got the vax had superior immunity to those without either, but it’s also possible for some the addition of the vax on top of prior infection did nothing.
If this is confusing, what it means is that this, or any, analysis that does not account for previous infection will overestimate, perhaps by a lot, vaccine efficacy. Because it will credit the vax for deaths that were prevented by previous infection. There is also the possibility that other “unapproved” treatments, such as vitamin D and ivermectin, which many took in 2021 also would give credit to the vax.
The authors then fit their same model to “excess” deaths. There are two major flaws with this.
The first is assuming all “excess” deaths were due to or related to covid. This is almost certainly false, as I analyze here. There were still large numbers of deaths above what was usually expected (that “usually expected” is a model) after subtracting covid deaths, especially early in the panic.
These could be caused by aggressive over-treatment for covid, lockdowns, and people too scared to have other life-threatening conditions checked out, especially in 2020 when the panic was at its peak.
The initial frenzied panic subsided somewhat in 2021, which means many “excess” deaths decreased merely from lessened panic. This, too, is credited to the vax in their model.
The second mistake, even allowing that all “excess” deaths were indeed covid related, is that the uncertainty in the estimates of “excess” deaths—there is a plus-or-minus for these—was not incorporated into the estimated number of lives saved by the vax. This means that their own plus-or-minus windows for lives saved will be far too narrow.
None of these criticisms mean that the vaccine did not offer some protection in some people, while also conferring some risk of injury from the vaccination itself. Our point is that there are massive uncertainties that were not accounted for, leading to an exaggerated view of the vaccine’s efficacy.
A further uncertainty is the global nature of this analysis. Countries varied widely in behavior to and in the panic. Vaccines differed. Background health differed. Official policy differed. Really, most things of important differed. Numbers are all over the map. A gross country-by-country analysis is leaving far too much uncertainty unaccounted for.
Especially because of the blind panic, and the failure to note prior infection, it will forever be difficult, or even impossible, to know just how efficacious the vaccine was in 2021.
I am a wholly independent writer, statistician, scientist and consultant. Previously a Professor at the Cornell Medical School, a Statistician at DoubleClick in its infancy, a Meteorologist with the National Weather Service, and an Electronic Cryptologist with the US Air Force (the only title I ever cared for was Staff Sergeant Briggs).
My PhD is in Mathematical Statistics: I am now an Uncertainty Philosopher, Epistemologist, Probability Puzzler, and Unmasker of Over-Certainty. My MS is in Atmospheric Physics, and Bachelors is in Meteorology & Math.
Author of Uncertainty: The Soul of Modeling, Probability & Statistics, a book which calls for a complete and fundamental change in the philosophy and practice of probability & statistics; author of two other books and dozens of works in fields of statistics, medicine, philosophy, meteorology and climatology, solar physics, and energy use appearing in both professional and popular outlets. Full CV (pdf updated rarely).
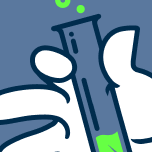
Support the Broken Science Initiative.
Subscribe today →
One Comment
Leave A Comment
You must be logged in to post a comment.
recent posts
Metabolic Flexibility to Burn Fat, Get Stronger, and Get Healthier
Expanding Horizons: Physical and Mental Rehabilitation for Juveniles in Ohio
This is my first time pay a quick visit at here and i am really happy to read everthing at one place