By R T. Cox
Summary
Probability theorists are primarily divided into two camps. Both are concerned with frequencies within a predictive framework and reasonable expectations. But each side prioritizes one over the other as the primary concept of probability. The frequency interpretation is more popular, but the author of this paper, Richard Cox believes this is a mistake. He provides the example of a box containing two white balls and one black ball, identical except for color. He then explains how each group would interpret this situation.
The frequentist interpretation would assess the probability of a white ball being drawn as ⅔. They solve the problem by imagining drawing one ball from an indefinite number of boxes; or repeatedly drawing one ball from a box and then replacing it. In either case, over a huge number of repetitions, the frequency of a white ball being drawn would converge at ⅔. According to them, this is not a prediction of probability theory, but a definition of probability. Probability is a characteristic of the “ensemble” (test setup) and doesn't exist without it.
In the second interpretation, probability theory provides the reasonable expectation of drawing a white ball two-thirds of the time and a black ball one-third of the time. This measure of reasonable expectation is the primary meaning of probability according to the second group.
The two interpretations of probability do have a lot in common. Both methods can algebraically calculate the probability that an event will occur or not, that one or another event will occur, or that both events will occur. Each also specifies that if an event is unlikely to occur, then it is more likely not to occur. Likewise, the probability of two events occurring together is less than the probability of the least likely of the two.
If frequencies and reasonable expectations were perfectly interchangeable, then the division among probability theorists would be purely grammatical. However, there are important differences. In some cases, it is impossible to imagine an ensemble that could repeatedly test the frequency of an event. But there are cases where a probability cannot be determined by an ensemble. For example, there is a notion in science that a simple hypothesis is preferred to a complex one. Two or more postulates being true is less likely than a single postulate of the same likelihood being true. This preference is a reasonable belief, but does not have a testable frequency.
Cox argues that the weakness of the frequentist interpretation is that there are so many situations where a definable ensemble does not exist. The rules that were derived from testing frequencies within ensembles cannot be justified in uses outside of this domain.
John Maynard Keynes developed an original theory of probability which does not depend on frequency. To Keynes, the theory of probability is a form of logic that applies to probable inferences. Probability is thus the degree of rational belief corresponding to a hypothesis and its conclusion. Probability excludes absolute certainty and impossibility (the domain of deductive logic), but concerns the values in between these extremes. The frequency definition of uncertainty is invalid because it treats probability as a property of an object which has a certain value, like distance or time in mechanics.
While Cox agrees with Keynes' views on probabilities, he felt there was still work to be done in defining its foundational axioms. Many of the postulates of Keynesian probability theory come from the study of games of chance (coin flips, dice, card games) and bear “tool marks” of this origin. Cox's goal was to derive foundational axioms of probability independent of any ensemble.
To do this, Cox outlines the basics of symbolic logic and provides eleven foundational axioms. Of these, six are foundational and the remaining five can be derived from the former. These axioms provide the basic rules by which algebraic manipulations can be performed on expressions of logical probability, which he defines next.
He uses the symbols b|a to describe the probability of b if a is accepted as true. He restates this as “the credibility of b on the hypothesis a.” Mathematical formulas can be used to operate on expressions of probability in this form. Cox demonstrates several, including multiplication and exponentials. The most important assumption of this section is that the probability of c and b given a (c·b|a) equals the probability of c given b and a and also b given a (c·b|a = c|b·a , b|a).
Cox explains this with an example of the probability that a runner can run a given distance and back on a certain track without stopping.
The value a is what we know about the physical condition of the runner and the track.
The value b is the evidence that he has run a given distance without stopping.
The value c is that he has returned from that distance without stopping.
The probability that the runner can run the first leg without stopping given what we know from a is: b|a. The probability that the runner returns without stopping given that the first leg was completed and also given what we know from a is: (c|b·a). Thus, the probability that the runner can run a certain distance and return (c·b|a) is a function of the first two probabilities.
A second assumption relates to the probability of b and not-b given a. The symbol, ~b, is used to mean “not b” in the sense that if b means “the car is white,” then ~b means “the car is not white.” The ~ symbol does not imply the contradiction, which would be “the car is black”. One important formula to know from this section is that the probability of b|a + ~b|a = 1. In other words, the sum of probabilities for b given a and not-b given a is 1 (a probability value of 1 means complete certainty).
Part one of this paper argued that probability has a wider scope beyond what the frequency definition implies. The second part derived the basic rules of probability from fundamental axioms. The third part of the essay explains how this new understanding of probability handles the frequency of an event.
Cox gives the example of two samples of radon. One is older than the other, but they are unlabelled. Each is attached to an identical ion counter and the goal is to predict which sample will reach 1000 ion counts first. A physicist with knowledge of quantum mechanics would estimate the two samples have equal probability of reaching 1000 counts first. An ordinary person without knowledge would derive the same probability, but out of ignorance. The estimate of the physicist is called an objective probability. The estimate of the non-physicist is commonly called a subjective probability, though Cox prefers the term “primary probability.” This term better describes a situation in which nothing is known about the problem.
Cox then describes a situation in which a die is rolled many times and may or may not have two sides with four dots. The frequency of rolling a four should be either 1 in 6 or one 1 in 3. In this case, a stable probability can never be reached, but it can be estimated. Cox then derives that an estimate grows “sharper” the more trials have been conducted. Though Cox doesn't use the term, this is often called “the Law of Large Numbers.” He then addresses an assumption of Laplace, that an unknown probability is equally likely to have any value between 0 and 1. This assumption is generally true, except in cases where the probability value is 0 or 1, or when the number of trials is very small. Cox proves that Laplace was correct, but with less generality than Laplace supposed.
Under Cox's reasonable expectation interpretation of probability, the frequency of an event approaches a stable probability as the number of instances increases and he concludes: “that is all we should expect of it.” A “true” frequency can never be reached, since an infinite number of experiments cannot be run.
Probability measures how much we know about an uncertain situation. There are two main ways to think about this. One way is to look at how often something happens if you repeat an experiment many times. The other way is to think about how reasonable it is to expect something will happen based on what you know. Most scientists believe the first way is correct, called “frequentism,” but this paper by Richard Cox says the second way is better.
To show how each opinion is different, the author used an example of picking a ball from a box with one white ball and two black balls. The first way would say that if you picked a ball out of this box many times, you would get a white ball one out of three times and that frequency of ⅓ is the probability. The second way would say it's reasonable to expect a white ball one out of three times. Even if you can only pick a ball once, it is still reasonable to judge this as the probability.
These two ways mostly agree on how to calculate probabilities. But there are some cases where the first way doesn't work because you can't repeat an experiment infinite times. One philosopher named John Maynard Keynes created a system to calculate probability based on logic (using rules to make judgments) instead of repeated experiments.
The author of this article wanted to come up with basic rules of probability for Keynes' system. He used symbols from math and logic to show probabilities. An example is “b|a” means "the probability of b if a is true." He shows how you can combine probabilities using math, like adding them together.
Cox also talks about how as you repeat an experiment more times, you get a better idea of the probability. He shows how the philosopher Laplace was right about this, but made some assumptions that weren't completely correct.
In the end, Cox says you can only approach the true probability of something by measuring its frequency. But there are a lot of other times you can't measure a frequency, but you can still make a reasonable guess.
--------- Original ---------
The concept of probability has from the beginning of the theory involved two ideas: the idea of frequency in an ensemble and the idea of reasonable expectation. The choice of one or the other as the primary meaning of probability has distinguished the two main schools of thought in theory.Probability theory is divided between two main perspectives. One is the frequency interpretation, which looks at the rate something occurs when repeating an experiment. The other is the reasonable expectation view, which considers how probable something is based on what you know. The author of this paper is Richard Cox, an American physicist. He argues that the frequency interpretation is wrong and makes a case for the reasonable expectation interpretation of probability.
The article provides the example of drawing a white ball from a box containing one white ball and two black balls. If you pick one ball from the box many times, you will pick a white ball 1 out of 3 times. So, the frequency side would say the probability of picking a white ball is ⅓. The reasonable expectation view would say it's sensible to expect drawing a white ball 1/3 of the time because ⅓ of the balls are white.
These outlooks often calculate probabilities similarly. But there are some ideas which have a probability, but can't be tested by repeated experiments. Philosopher John Maynard Keynes saw probability as something that could be expressed using logic (rules of how ideas relate to one another).
The author of this paper wanted to create basic rules of probability, called axioms, to go with Keynes' philosophy. He demonstrated that there are 11 fundamental axioms and six of them can be created from the first five. He also taught how symbols like “b|a” can be used to mean "probability of b if a is true." Then he demonstrated how those symbols representing probabilities could be manipulated with math operations.
Cox also examined an idea, sometimes called “the Law of Large Numbers,” which says how an estimate of probability gets more accurate after an experiment is repeated many times. He showed that the philosopher Laplace was right about this, but made some incorrect assumptions.
In the end, Cox says that an estimate of the probability of something becomes more stable after many measurements, but this is the most you can say. So, the frequency interpretation of probability can never reach a true probability frequency because you can never run an infinite number of experiments. And there are lots of times you can't even run multiple experiments, so the reasonable expectations interpretation makes more sense in more situations.
--------- Original ---------
The concept of probability has from the beginning of the theory involved two ideas: the idea of frequency in an ensemble and the idea of reasonable expectation. The choice of one or the other as the primary meaning of probability has distinguished the two main schools of thought in theory.Let's start with the truth!
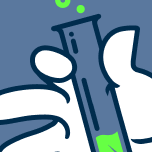
Support the Broken Science Initiative.
Subscribe today →
recent posts
Jaynes foresaw reasoning machines aiding human inquiry, but modern AI's misuse, such as in academic fraud, underscores the necessity of scientific rigor.
As reported by the BBC, Bartlett’s podcast “is amplifying harmful health misinformation on his number-one ranked podcast.”